Runpod vs Paperspace: Which Cloud GPU Service Delivers the Best Performance?
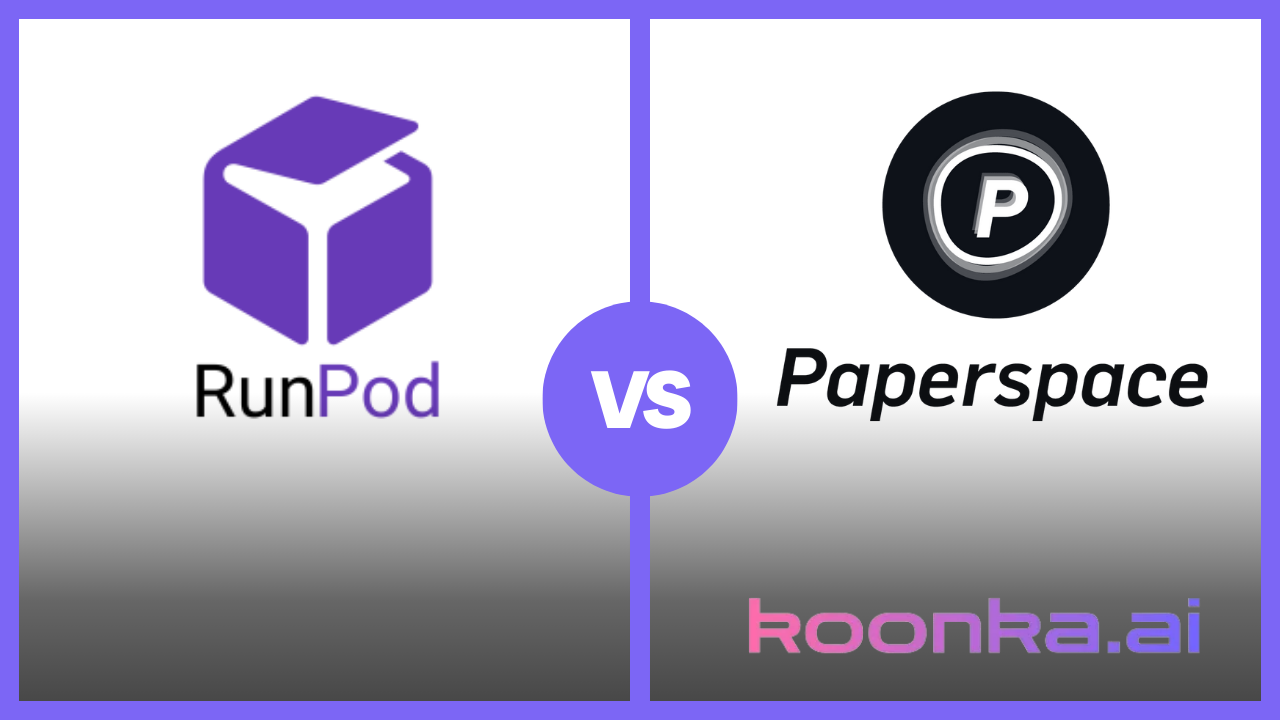
Choosing the right cloud GPU platform can make or break your AI project. Whether you’re deploying machine learning models or running heavy computations, you need a service that balances performance, ease of use, and cost. That’s where Runpod and Paperspace come in. Both promise powerful GPUs, lightning-fast performance, and scalable solutions. But how do they really stack up? We’ll dive deep into Runpod vs Paperspace, comparing their features, pricing, and performance. By the end, you’ll know which one is the best fit for your AI workloads, without all the fluff.
Affiliate Disclosure
We prioritize transparency with our readers. If you purchase through our affiliate links, we may earn a commission at no additional cost to you. These commissions enable us to provide independent, high-quality content to our readers. We only recommend products and services that we personally use or have thoroughly researched and believe will add value to our audience.
Table of Contents
Cloud GPU Comparison
Choosing between RunPod and Paperspace can be overwhelming, especially if you’re deciding which cloud GPU provider will serve your AI, ML, or any other GPU-based workloads best. Both providers excel in different areas, and understanding their strengths will help you choose the one that fits your needs.
Looking for affordable, high-performance GPUs? CUDO Compute lets you rent top-tier NVIDIA and AMD GPUs on-demand. Sign up now!
To learn more about CUDO Compute, kindly watch the following video:
GPU Models and Architecture
Both RunPod and Paperspace offer a range of modern graphics processing units (GPUs), primarily based on NVIDIA and AMD architectures. Let’s break down the core options:
RunPod GPU Options:
RunPod offers a comprehensive range of GPUs suitable for various computing workloads. Notably, RunPod offers NVIDIA’s H100 PCIe at $2.69/hour and A100 PCIe at $1.19/hour. These GPUs are highly regarded for advanced cloud acceleration, enabling both AI training and inference workloads at scale. RunPod also includes budget-friendly options like the A40 starting at $0.39/hour, offering excellent price-to-performance ratios for smaller tasks.
Some Standout models include:
NVIDIA A100 (Starting at $1.19/hour)
The NVIDIA A100 is a powerhouse in the world of GPUs, designed primarily for AI and data-heavy tasks. It’s built on the Ampere architecture, which brings improved performance and efficiency. This GPU is ideal for deep learning, machine learning, and large-scale data processing, offering unmatched parallel processing power. It comes with 40 GB or 80 GB of HBM2e memory, making it capable of handling massive datasets with ease.
One of its key features is multi-instance GPU (MIG) technology, which allows the A100 to be partitioned into several smaller GPUs. This makes it highly efficient for multi-tenant environments, where different users or tasks can share the GPU without performance issues. If you’re running complex AI models or processing huge datasets, the A100 provides fast training times and excellent scalability.
While it’s more expensive to rent, the value you get from its high-end performance justifies the cost, especially for large projects where time savings are crucial. If you need top-tier power for enterprise-level AI or data science work, the NVIDIA A100 is an excellent choice, despite its higher price per hour.
NVIDIA RTX 4090 (Starting at $0.44/hour)
The NVIDIA RTX 4090 is a consumer-grade GPU, but don’t let that fool you—it’s a beast for gaming, 3D rendering, and even some AI work. Built on the Ada Lovelace architecture, it offers a huge leap in performance compared to previous generations. With 24 GB of GDDR6X memory, it can handle highly detailed 3D graphics, ray tracing, and demanding computational tasks with ease.
The RTX 4090 shines in creative workflows, such as video editing, animation, and 3D modeling, thanks to its superior CUDA core count and ray-tracing capabilities. It’s also a popular choice for gamers who want top-tier performance at high resolutions like 4K. While it’s not specifically built for AI and data science tasks, it can still handle them fairly well, making it a versatile GPU for those who need a bit of everything.
At $0.44/hour, it offers incredible value for people who need a powerful, multi-purpose GPU without breaking the bank. For professionals and gamers alike, the RTX 4090 delivers a high-performance punch at a reasonable price, making it a solid choice for both gaming and creative applications.
AMD MI300X (Starting at $3.99/hour)
The AMD MI300X is a cutting-edge GPU designed for AI and high-performance computing (HPC). It’s part of AMD’s Instinct series, targeting enterprise-level workloads like deep learning, AI training, and data analytics. What sets the MI300X apart is its use of the CDNA 3 architecture, which focuses on AI and machine learning tasks with exceptional efficiency. It offers up to 128 GB of HBM3 memory, allowing it to handle the largest AI models and datasets with ease.
One of the MI300X’s standout features is its ability to integrate both CPU and GPU cores on a single chip. This design reduces data transfer bottlenecks between the CPU and GPU, offering faster processing times and more efficient workflows. It’s especially useful for AI models that need a lot of memory and computational power.
Although the MI300X comes at a higher price point of $3.99/hour, it’s ideal for companies or researchers who need the absolute best in performance and scalability for massive AI workloads. Its efficiency and sheer power make it a strong competitor to NVIDIA’s top-tier GPUs, especially in tasks that require massive parallel processing and memory capacity.
RunPod allows users to choose from 50+ templates, or configure their own environment using popular frameworks like PyTorch or TensorFlow.
Boost your AI and machine learning workloads with CUDO Compute’s powerful cloud GPUs. Start scaling today. Sign up now!
Paperspace GPU Options:
Paperspace also features a broad selection of GPUs, tailored for machine learning and data-heavy tasks. They offer competitive pricing with the NVIDIA H100 available for $5.95/hour and the NVIDIA A100 at $1.15/hour. Their GPUs range from entry-level to advanced, including the A4000 at $0.76/hour, perfect for smaller projects.
Some popular options include:
NVIDIA A100 (Starting at $1.15/hour)
The NVIDIA A100 is a top-tier GPU, designed for AI, machine learning, and high-performance computing (HPC). Built on the Ampere architecture, it delivers incredible parallel processing capabilities, making it perfect for deep learning models and large-scale data analytics. It comes with 40 GB or 80 GB of HBM2e memory, which allows it to handle massive datasets and complex AI models without breaking a sweat.
One of its standout features is the multi-instance GPU (MIG) technology, which enables the A100 to be split into multiple smaller GPUs. This is particularly useful for environments where multiple users or tasks need to share the same GPU without compromising performance. It offers immense computational power, ideal for speeding up training times for AI models and improving the overall efficiency of large data workflows.
While it’s more expensive than some other GPUs, the A100’s power justifies the cost for enterprise-level AI projects. If you’re working on deep learning, AI training, or massive data tasks, this GPU can significantly reduce processing time and offer the scalability needed for high-demand workloads.
NVIDIA A6000 (Starting at $1.89/hour)
The NVIDIA A6000 is another high-performance GPU, available through Google Cloud Platform, that caters to professionals needing extreme computational power. Built on the Ampere architecture, it boasts 48 GB of GDDR6 memory, making it perfect for tasks like 3D rendering, AI development, and large-scale simulations.
While it’s slightly more expensive than the A100, the A6000 excels in creative workflows such as rendering and 3D modeling, in addition to AI tasks. When combined with other Google Cloud services, this GPU is ideal for creative professionals who require top-notch performance in fields like architecture, visual effects, and video production. The A6000 is a strong option for those needing flexible, cloud-based access to high-end computing without the upfront costs of physical hardware.
In cloud environments, the A6000’s efficiency makes it great for maximizing bandwidth pooling servers, especially when scaling complex creative or computational tasks. If you’re using a cloud provider like Google Cloud for tasks that demand both precision and speed, the A6000 offers unmatched versatility.
NVIDIA RTX 5000 (Starting at $0.82/hour)
The NVIDIA RTX 5000 is a solid mid-range GPU designed for professionals in industries like design, architecture, and AI. Built on the Turing architecture, it comes with 16 GB of GDDR6 memory, making it capable of handling moderately complex workloads. It’s often used for tasks like 3D modeling, video editing, and AI inference, providing a good balance between price and performance.
While not as powerful as the A100 or A6000, the RTX 5000 still packs a punch, especially in rendering and AI workflows. Its ray-tracing capabilities are well-suited for realistic lighting in visual projects, and it has strong CUDA core support for machine learning and AI applications. This GPU is ideal for professionals who need reliable performance without the price tag of top-tier GPUs.
At $0.82/hour, the RTX 5000 offers great value for those who need a capable, reliable GPU but don’t require the extreme power of more expensive models. It’s perfect for small to mid-sized projects where performance is important, but budget is a consideration, making it a popular choice for designers, architects, and researchers working on AI models.
Both RunPod and Paperspace support the latest state-of-the-art GPUs, including multi-GPU configurations, making them excellent choices for independent software vendors who need flexibility.
Need flexible, scalable GPU cloud services for AI or rendering? CUDO Compute offers reliable options at great prices. Sign up now!
Pricing and Cost-Effectiveness
When it comes to cost, both RunPod and Paperspace provide clear and predictable pricing models. However, there are some distinctions that can make a difference based on your budget and use case.
RunPod Pricing:
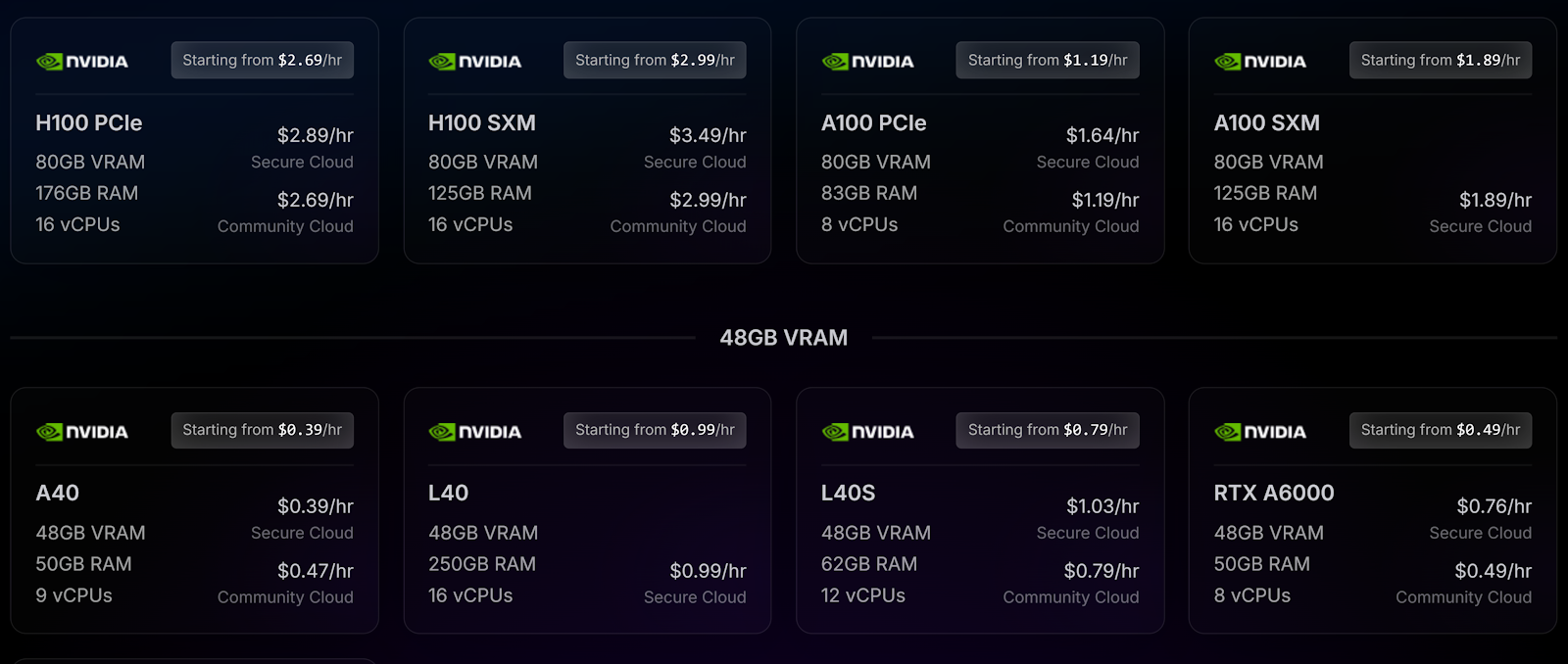
RunPod shines in offering affordable GPU computing options. Its pricing is on a per-minute basis, so you pay only for the time you use. Their RTX A5000, for example, starts at $0.22/hour, making it a great option for those who want cost-effective cloud GPU services.
For large-scale operations, you can save up to 15% with serverless offerings like their A100 GPU, where the flex price is $0.00060/second. This makes RunPod ideal for scaling GPU workloads with minimal overhead.
RunPod’s pricing for different GPUs:
- A40: $0.39/hour
- A100 SXM: $1.94/hour
- MI300X: $3.99/hour
Paperspace Pricing:
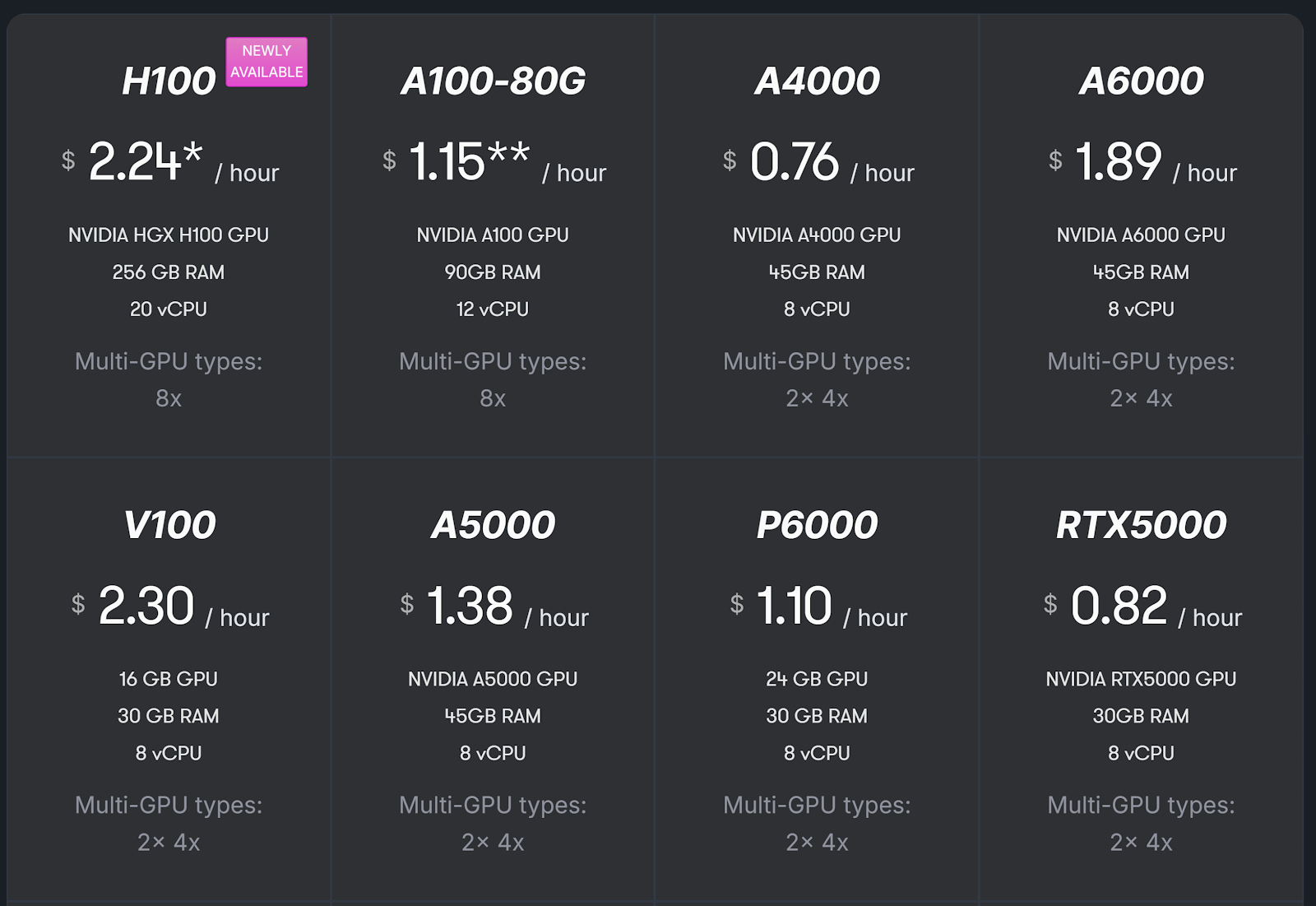
Paperspace, too, offers predictable costs but differs in that its H100 on-demand pricing is higher at $5.95/hour. On the lower end, their RTX 4000 starts at $0.56/hour and can go up to $2.24/hour for the H100 with a 3-year commitment.
Paperspace’s pricing breakdown:
- A100-80G: $1.15/hour
- RTX 4000: $0.56/hour
- H100 (On-Demand): $5.95/hour
Both providers offer per-second billing, meaning users only pay for actual usage time, making both platforms cost-efficient.
Get instant access to NVIDIA and AMD GPUs on-demand with CUDO Compute—perfect for AI, ML, and HPC projects. Sign up now!
Cloud-Based Virtual Machines and Instance Customization
RunPod:
RunPod’s cloud-based virtual machines are easily customizable. You can choose from community or secure cloud offerings, or bring your own container. It provides dedicated bare metal servers and persistent network storage, making it easier to manage your cloud infrastructure with zero operational overhead.
For those needing dedicated resources, RunPod Secure Cloud supports GPU instances with guaranteed availability, perfect for demanding computing infrastructure tasks.
Paperspace:
Similarly, Paperspace offers on-demand compute with flexible configuration options. Their dedicated GPUs allow for root access via SSH, which is ideal for teams needing granular control over their environment. With their multi-GPU types, Paperspace also supports larger, high-performance projects.
Paperspace’s multi-GPU instances are available in 2X, 4X, and 8X variants, catering to more complex workloads.
Performance and Networking
Performance is a key factor in cloud computing, and both platforms deliver on speed and reliability.
RunPod:
RunPod’s globally distributed node infrastructure ensures high-speed data transfer and low-latency performance across multiple regions. They provide strategic edge locations to reduce latency and improve performance. Additionally, RunPod Secure Cloud supports bandwidth pooling, so tasks are handled more efficiently.
RunPod also promises lightning-fast networking with up to 100Gbps network throughput, which is ideal for AI inference and training large models like GPT or Llama. Their serverless GPUs also autoscale in seconds, allowing you to respond to real-time user demand efficiently.
Paperspace:
Paperspace offers lightning-fast networking with each instance connected to a 10 Gbps backend network. Their Windows server compatibility allows for a broad range of workloads, ensuring globally distributed node infrastructure to power ML or gaming workloads with minimal lag.
Paperspace’s dedicated bare metal servers and flexible configuration options ensure high reliability and speed, backed by their strategic edge locations.
CUDO Compute delivers top-tier performance and cost-effective cloud GPU solutions, designed to power your AI and ML tasks. Sign up now!
Managed Services and Usability
RunPod:
RunPod offers a highly streamlined managed service experience, especially for those looking to reduce infrastructure management headaches. Their platform supports managed service providers and lets users scale effortlessly with autoscaling and serverless offerings.
The RunPod CLI provides a seamless development experience, allowing for quick deployments and testing via runpodctl. Their hot-reload feature and real-time logs give you immediate feedback on your deployments.
Paperspace:
Paperspace’s simple management interface makes it user-friendly for teams of all sizes. It comes preloaded with machine learning frameworks, and its API allows for easy integration and scaling of tasks. Paperspace ML in a Box simplifies deployment by providing pre-installed environments for ML development.
For users needing GPU instances with full control, Paperspace provides root access and SSH key integration, making it a robust option for experienced developers.
Strategic Edge Locations and Global Reach
RunPod:
With 30+ regions globally, RunPod ensures that workloads are handled in proximity to users, minimizing latency and improving cloud-based virtual machines performance. Their strategic edge locations allow faster data transfer and improved uptime.
Paperspace:
Paperspace also offers globally distributed node infrastructure, ensuring low-latency and reliable connections for any project. Their strategic edge locations make them a solid option for cloud GPU providers working across multiple regions.
Key Use Cases
Both RunPod and Paperspace excel in different areas:
RunPod:
- AI Inference and Training: RunPod’s support for serverless and scalable infrastructure makes it an excellent choice for AI training and inference workloads.
- Enterprise Use: With secure, dedicated instances and comprehensive logging, RunPod caters well to enterprise clients needing stable, secure cloud environments.
Paperspace:
- Machine Learning Development: Paperspace’s preconfigured ML environments simplify independent software vendors and startups’ workflows, offering flexible compute with minimal setup time.
- Flexible Scaling: For users looking to scale effortlessly, Paperspace’s multi-GPU instances provide the flexibility needed for heavy computing tasks.
Runpod vs Paperspace: Which Cloud GPU Provider Should You Choose?
When deciding between RunPod and Paperspace, consider your project needs and budget.
RunPod excels in offering cost-effective cloud GPU services with affordable entry-level GPUs, making it ideal for startups, researchers, or projects that need high performance at a lower price.
Paperspace stands out with its ease of use and quick setup, making it an excellent choice for those needing high-end GPUs and more advanced management features, especially for machine learning projects.
Both cloud GPU providers offer excellent services, but your specific needs in terms of pricing, scalability, and GPU models will help you make the right decision.
Ready to level up your computing power? Try CUDO Compute’s flexible cloud GPUs and launch an instance in minutes. Sign up now!