RunPod vs Azure VM: GPU Cloud Services for AI – A Detailed Comparison
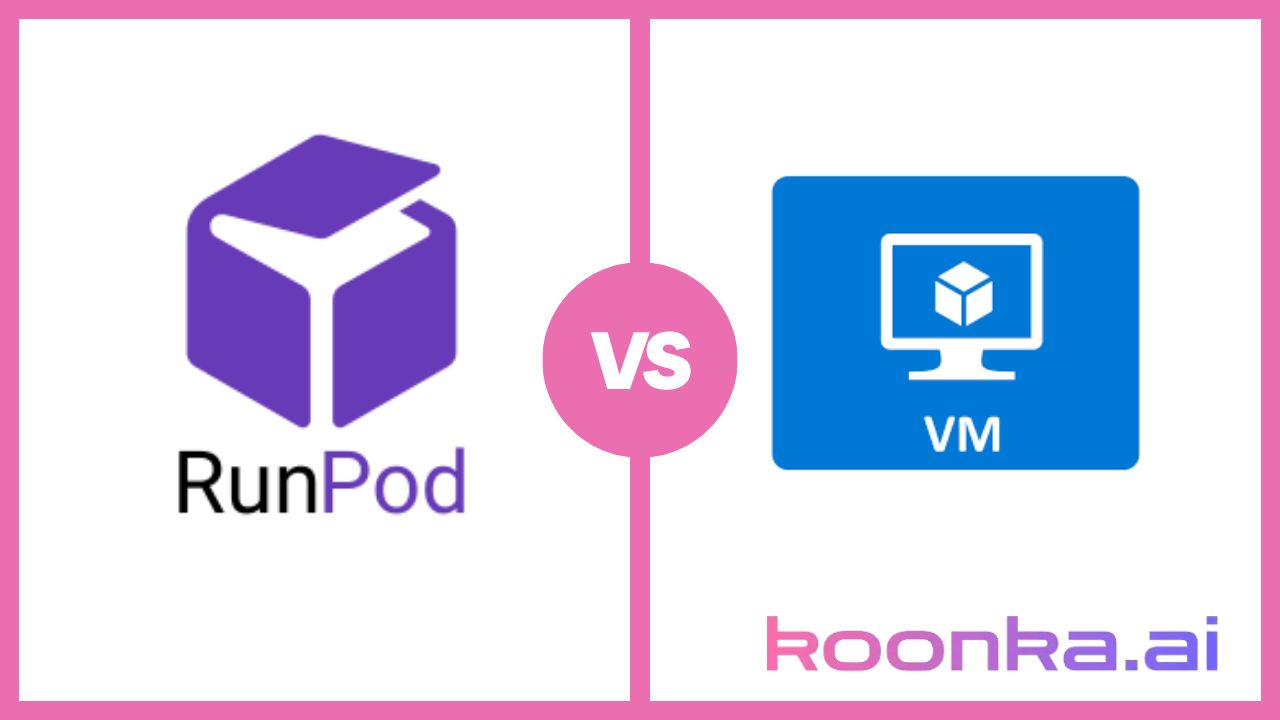
If you’re working on AI or machine learning, the choice of cloud provider for GPU services is key. Two big players you’ll come across are RunPod and Azure VM. Each has its pros, from super-fast cold starts to global scale. RunPod promises seamless deployment and instant spin-up, designed for heavy AI workloads. Azure VM has a ton of GPU-enabled options, backed by Microsoft’s infrastructure and enterprise-grade security. Both have competitive GPU cloud options so knowing the details will help you decide which is best for you – especially when it comes to GPU models and pricing. Let’s get into the key differences.
Affiliate Disclosure
We are committed to being transparent with our audience. When you purchase via our affiliate links, we may receive a commission at no extra cost to you. These commissions support our ability to deliver independent and high-quality content. We only endorse products and services that we have personally used or carefully researched, ensuring they provide real value to our readers.
Table of Contents
RunPod vs Azure Virtual Machines: An Overview
Feature | RunPod | Microsoft Azure VM |
Top GPUs | H100, A100, RTX 4090 | H100, A100, P100, V100, T4 |
Pricing (Hourly) | $0.44 to $3.49 | $0.53 to $14.69 (depends on GPU and configuration) |
Storage Pricing | $0.07 to $0.10/GB/month | Based on tier (Blob storage) |
Use Case Fit | AI/ML workloads, rendering, affordable scaling | Large-scale AI, big data analytics, enterprise solutions |
Network Performance | Pod and network storage options | InfiniBand for fast interconnect in multi-GPU instances |
Scalability | High scalability at affordable prices | Enterprise-level scalability, global infrastructure |
CUDO Compute offers cost-effective, high-performance GPUs, perfect for AI, ML, and HPC workloads, starting from as low as $0.30/hr. Sign up now!
For a deeper understanding of CUDO Compute, take a look at this informative video:
RunPod vs Azure VM: GPU Models and Pricing
RunPod GPU Models and Pricing
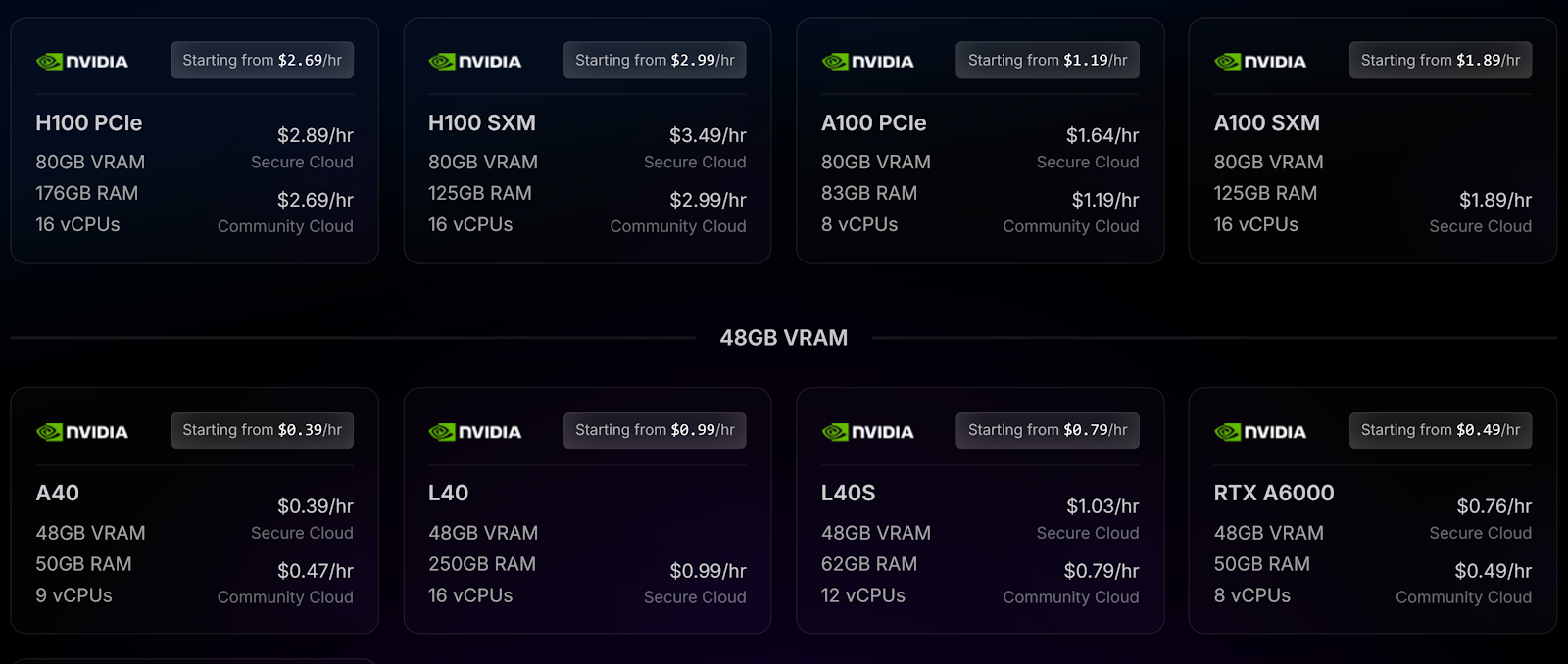
RunPod offers a wide array of GPU models with competitive pricing, structured by hourly rates and split into Secure Cloud and Community Cloud environments. The GPUs are billed by the minute, with no ingress/egress fees, making it a cost-effective solution for users with fluctuating workloads.
MI300X (AMD, 192GB VRAM)
- Price: $3.49/hr
- Use Cases: Best suited for large-scale AI models, HPC, and data analytics due to its high VRAM and computing power.
H100 (NVIDIA, 80GB VRAM, PCIe)
- Price: $2.69/hr (Secure Cloud), $2.69/hr (Community Cloud)
- RAM: 188GB, 16 vCPUs
- Use Cases: Ideal for users running high-performance machine learning and AI inference tasks, such as LLMs and deep learning frameworks.
H100 (SXM)
- Price: $2.99/hr
- RAM: 125GB, 16 vCPUs
- Use Cases: Excellent for machine learning and deep learning model training requiring larger memory and faster connectivity than PCIe.
A100 (NVIDIA, 80GB VRAM)
- Price: $1.64/hr (Secure Cloud), $1.19/hr (Community Cloud)
- RAM: 83GB, 8 vCPUs
- Use Cases: Targeted for high-throughput deep learning tasks like GPT models, but more affordable than H100 models.
L40 (NVIDIA, 48GB VRAM)
- Price: $0.99/hr
- RAM: 125GB, 16 vCPUs
- Use Cases: Perfect for rendering, 3D workloads, and general high-performance computing that requires an excellent balance between price and performance.
RTX 4090 (NVIDIA, 24GB VRAM)
- Price: $0.69/hr (Secure Cloud), $0.44/hr (Community Cloud)
- RAM: 26GB, 4 vCPUs
- Use Cases: Great for consumer-level deep learning, training smaller models, or prototyping before scaling up to more powerful GPUs.
RTX 3070 (NVIDIA, 8GB VRAM)
- Price: $0.13/hr (Community Cloud)
- RAM: 14GB, 4 vCPUs
- Use Cases: Cost-effective for light ML tasks, media encoding, and gaming workloads.
Azure GPU Models and Pricing
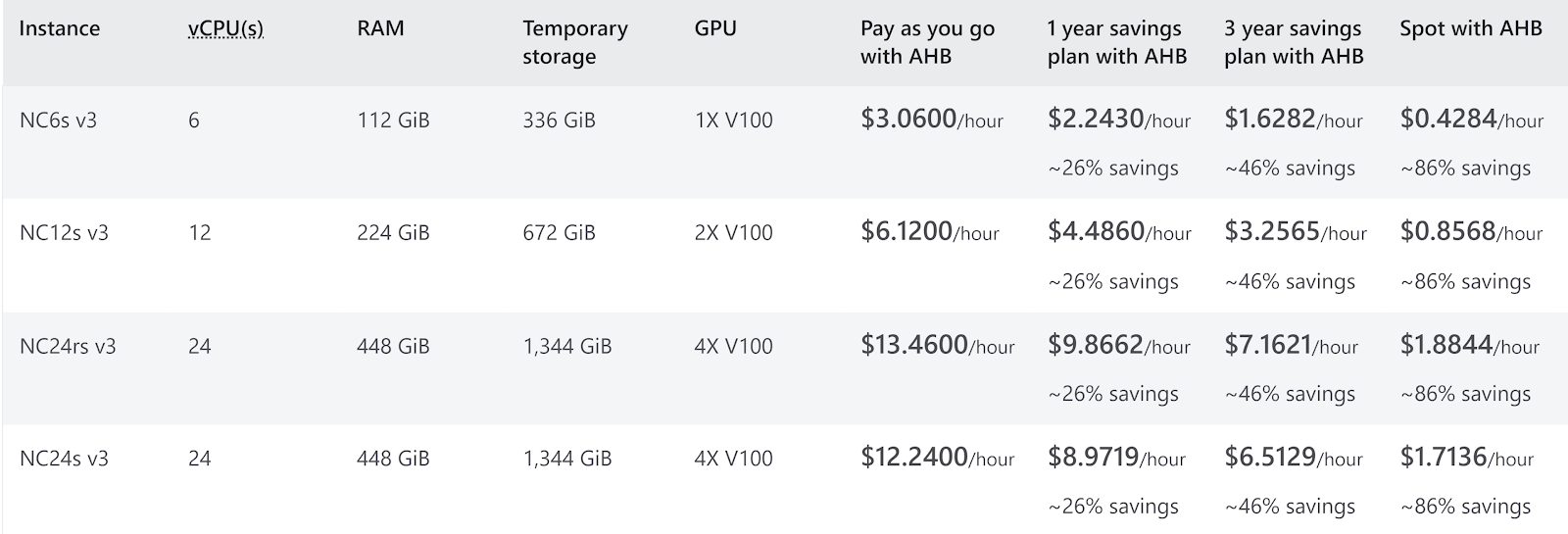
Microsoft Azure’s GPU instances are built for a wide variety of tasks, including machine learning, graphics rendering, and AI. The platform offers significant flexibility through different savings plans, including Pay-as-You-Go, 1-year, 3-year, and Spot instances.
H100 NVL (94GB VRAM)
- Price: $6.98/hr (Pay as you go)
- RAM: 320 GiB (40 vCPUs for NC40ads H100)
- Use Cases: The H100 series is highly optimized for large-scale AI training and inference, making it ideal for users running advanced language models or image processing tasks.
A100 (80GB VRAM)
- Price: $3.67/hr for 1x A100, $14.69/hr for 4x A100 (Pay as you go)
- RAM: 220 GiB to 880 GiB, depending on the instance type.
- Use Cases: Suited for massive AI workloads, deep learning, and parallel computing due to the sheer compute power and flexibility in scaling across different instance configurations.
P100 (16GB VRAM)
- Price: $2.07/hr (1x P100), $9.11/hr (4x P100)
- RAM: Up to 448 GiB
- Use Cases: Popular for high-performance computing tasks, including reservoir modeling, Monte Carlo simulations, and molecular dynamics.
V100 (16GB VRAM)
- Price: $3.06/hr (1x V100), $12.24/hr (4x V100)
- RAM: Up to 448 GiB
- Use Cases: Useful for large-scale ML training, and users can take advantage of multiple GPU instances with fast interconnect via InfiniBand for tasks like genome sequencing or financial simulations.
T4 (16GB VRAM)
- Price: $0.53/hr for NCas_T4 (Pay as you go)
- RAM: 28 GiB to 440 GiB depending on instance size
- Use Cases: Perfect for inference, smaller AI models, and VDI workloads. Affordable yet efficient for users looking to balance cost and performance.
Deploy powerful GPUs like H100 and A100 for your AI workloads through CUDO Compute, with prices starting at $1.59/hr. Sign up now!
RunPod vs Azure VM: Performance and Efficiency
RunPod Performance
RunPod has a wide range of NVIDIA and AMD GPUs for various workloads. From the high-end H100 and A100 to consumer-grade GPUs like the RTX series, RunPod has a GPU for your use case. Performance is coupled with flexible pricing models so it’s great for workloads that fluctuate or need on-demand scaling.
H100 and A100:
The H100 and A100 models on RunPod cater to large-scale AI tasks. They are particularly useful for researchers and developers working with large language models (LLMs) such as GPT, where model training can be accelerated significantly by using these GPUs.
L40 and L40S:
The L40 is optimized for high-performance inference tasks, and it shines in LLMs like LLaMA 3. It delivers strong inference throughput at a more affordable price compared to the H100, making it a go-to for users scaling down inference tasks from high-end GPUs.
RTX 4090 and 3090:
These GPUs provide an affordable option for deep learning tasks at the consumer level. Their strong GPU memory bandwidth and tensor cores make them ideal for training smaller machine-learning models before moving to larger-scale GPUs.
Azure Performance
Microsoft Azure has GPU instances for compute-intensive workloads. Configurations with high VRAM (e.g. H100 with 94GB of VRAM) and high memory configurations (up to 880 GiB RAM in NC96ads A100 v4) for large-scale workloads.
NCads H100 v5 Series:
This series delivers unparalleled performance for machine learning models that need vast amounts of VRAM, such as transformers used in natural language processing (NLP). For large enterprises that handle large datasets or require distributed computing, this series is a top choice.
NC A100 v4 Series:
Microsoft Azure’s A100 instances are optimized for AI and machine learning workloads that require multi-GPU configurations. With InfiniBand for fast interconnect and high memory availability, it’s ideal for use cases like genome sequencing, drug discovery, and other scientific computing workloads.
NCsv2 and NCsv3 Series:
These series leverage older NVIDIA GPUs like the P100 and V100, which still offer excellent performance for traditional HPC tasks and moderate deep learning models. The flexibility in instance configuration makes it suitable for scaling up or down based on workload intensity.
With CUDO Compute, you can run advanced AI models like Google Gemma or deploy PyTorch on the latest Ampere architecture GPUs. Sign up now!
RunPod vs Azure VM: Storage and Network Throughput
When comparing cloud GPU providers, RunPod and Microsoft Azure stand out for their different approaches to storage and network throughput. Both have powerful infrastructure for users working with big data, AI/ML workloads, and video streaming solutions. But they differ a lot in pricing model, storage flexibility, and network performance.
RunPod Storage and Network
RunPod has a very flexible and scalable storage model where you can choose between persistent storage and temporary storage. This flexibility is key for users who need to process big data without incurring unnecessary costs for idle storage. RunPod’s network storage pricing starts at $0.07/GB/month for volumes under 1TB and goes down to $0.05/GB/month for storage over 1TB. So RunPod is a great choice among cloud GPU providers for users who care about cost and scalability.
Pod Storage:
RunPod’s pod volumes and container disk pricing are based. Running pods are $0.10/GB/month and idle pods are $0.20/GB/month. This way you only pay for what you use. This is especially useful for users who need enterprise-level data security while working on AI/ML tasks or video streaming.
Persistent Network Storage:
Persistent network storage is required for handling big data in machine learning and big data analytics. RunPod’s private cloud network resources provide high-speed and secure transfer of business-critical data and seamless integration for tasks that require constant access to big data. This also supports traditional file transfer software solutions so data flows smoothly even for the most data-intensive applications.
Azure Storage and Network
Azure has an enterprise-focused storage and network solution that can scale to exabytes of data across its global network. Azure has a full ecosystem of Azure Blob Storage, data lakes, and integrations with services like CosmosDB, SQL Server, and other Google Cloud services. For enterprises, this is a top-tier solution as it has robust enterprise management capabilities and seamless integration with AI/ML tools, cloud-based databases, and secure data storage solutions.
Azure Blob Storage:
For users pairing GPU instanc TVes with storage, Microsoft Azure has Blob Storage which has flexible pricing based on redundancy levels and storage tiers. This is great for users working with large AI models as it gives immediate access to large data lakes that support secure virtual access. Plus Azure blob storage simplifies backups and data integrity so it’s ideal for enterprises that require enterprise-level data security.
High Throughput and InfiniBand:
Azure’s NC24rs v3 instances with InfiniBand have extremely high throughput so users can do distributed model training and big data analytics without performance bottlenecks. This type of connectivity is critical for companies that do secure business critical transfers of large datasets. Plus Azure’s SQL protection simplifies backups and gives extra protection for data-intensive workloads, especially in AI/ML and big data.
CUDO Compute provides enterprise-grade solutions, allowing you to reserve GPUs, deploy clusters, and scale effortlessly to meet your needs. Sign up now!
Which Cloud Solution Excels in Storage and Network Performance?
Both RunPod and Microsoft Azure have robust cloud infrastructure but their approach to storage and networking is different depending on your needs. If you want flexible, scalable, and cost-effective storage, RunPod stands out with pay-per-use pricing and private cloud network resources with video streaming solutions supported.
It’s great for dynamic workloads and secure access as it integrates OpenVPN server capabilities and supports OpenVPN client software packages. You can also use RunPod for specific workloads like AI/ML training as a cloud GPU provider, it’s a versatile option for many use cases as the OpenVPN Access Server supports video streaming solutions.
But Microsoft Azure like Oracle Cloud and IBM is for enterprises that need enterprise-level data security and scalable storage and networking. Microsoft Azure has InfiniBand connectivity and seamless integration with other cloud services. Its high-performance offerings are great for heavy workloads like AI/ML and real-time data processing.
Microsoft Azure Virtual Machines can also be used for specific use cases like high-throughput and low-latency storage for real-time video streaming tasks (called Ant Media Server). Paired with other Google Cloud services this makes Microsoft Azure a strong contender for enterprise needs.
Ultimately, the choice depends on your operational scale and the level of storage and network performance required for your specific use cases.
RunPod vs Azure VM: Which one should you go for?
Choosing between RunPod and Microsoft Azure for GPU instances depends on your specific needs in terms of pricing, performance, and use case.
- Go for RunPod if cost-efficiency and flexibility are your priorities. It’s ideal for individual developers, startups, or small teams needing access to high-performance GPUs like the H100 or A100 at competitive prices. With its pay-by-the-minute pricing model and no egress fees, RunPod is perfect for AI/ML model training, prototyping, and scalable workloads. It also offers more affordable GPUs, such as the RTX 4090, for lighter workloads or for those testing smaller models before scaling. If you need flexible, on-demand access to GPUs without long-term commitments or enterprise-level needs, RunPod is the better choice.
- Go for Microsoft Azure Virtual Machines if you’re an enterprise or large-scale organization needing robust infrastructure, enterprise-grade support, and global scalability. Microsoft Azure’s offerings, especially the H100 and A100, are optimized for large-scale AI models, big data analytics, and high-performance computing (HPC). Microsoft Azure’s InfiniBand connectivity and distributed storage make it ideal for workloads requiring vast data processing power. While it’s more expensive, Microsoft Azure Virtual Machines offer long-term discounts through savings plans, making it a smart investment for long-term, large-scale projects.
So, choose RunPod for cost-effective, flexible usage and Microsoft Azure for enterprise-level scalability and advanced AI workloads.
Choose from top-performing NVIDIA and AMD GPUs with CUDO Compute to supercharge deep learning, data science, and rendering tasks. Sign up now!