Replicate vs Fal.ai: Cloud GPU Models for AI Projects Compared
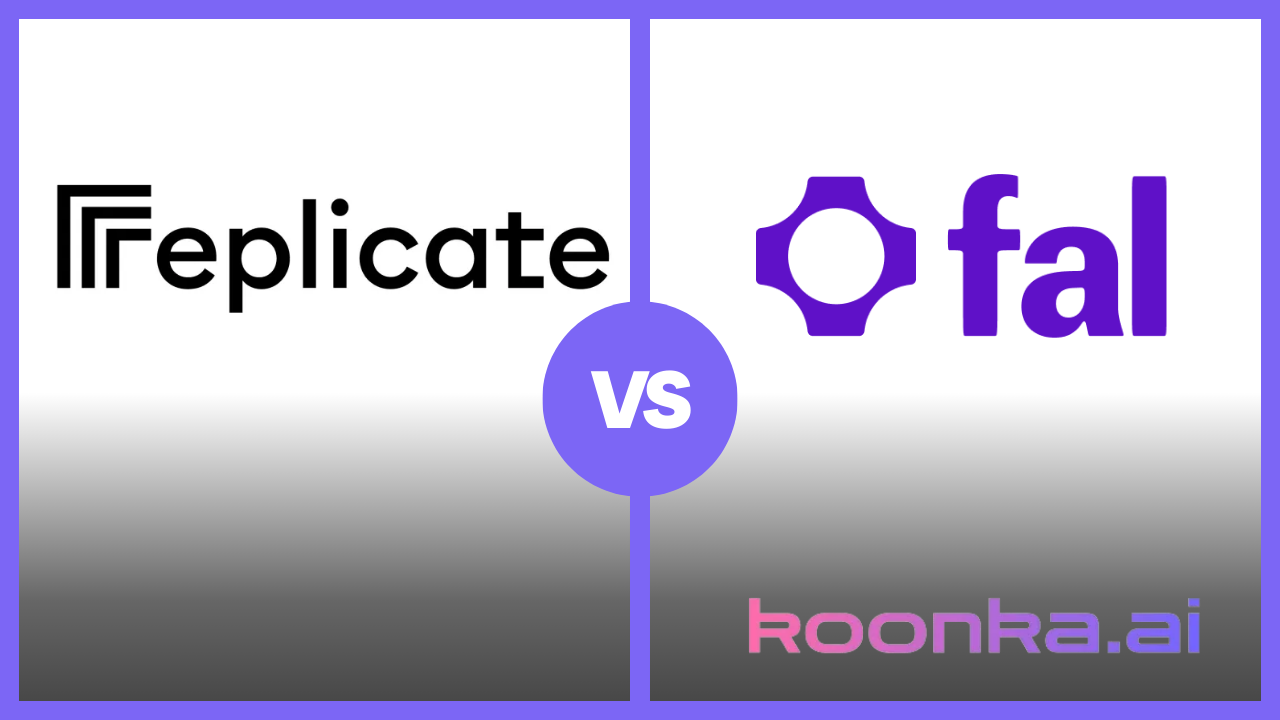
In the battle of Replicate vs Fal.ai, choosing the right Cloud GPU can make all the difference for your AI projects. Both platforms offer solid GPU options, but they each have their own strengths. Whether you’re running massive models or just need something quick and efficient, understanding how they stack up in terms of pricing, performance, and scalability is key. We’re breaking down the Cloud GPU models from Replicate and Fal.ai, so you can easily figure out which one’s the better fit for you.
Affiliate Disclosure
We prioritize transparency with our readers. If you purchase through our affiliate links, we may earn a commission at no additional cost to you. These commissions enable us to provide independent, high-quality content to our readers. We only recommend products and services that we personally use or have thoroughly researched and believe will add value to our audience.
Table of Contents
Cloud GPU Comparison
Both Replicate and Fal.ai offer cloud-based GPU services, but each has its own approach to performance, pricing, and overall value. Whether you’re deploying machine learning models, generating images, or fine-tuning existing models, selecting the right GPU setup can save you time, money, and frustration.
In this section, we’ll dive deep into the Cloud GPU models offered by Replicate and Fal.ai. We’ll break down everything from the specific hardware, pricing, and use cases to help you choose the best platform for your needs.
Need budget-friendly, high-performance GPUs? CUDO Compute offers on-demand rentals of premium NVIDIA and AMD GPUs. Sign up now!
To learn more about CUDO Compute, kindly watch the following video:
Overview of GPU Models
Before we dive into specifics, let’s get a quick overview of the GPUs each platform offers.
Replicate’s Cloud GPU Models
Replicate offers a range of GPU options, designed to scale based on the complexity of your AI models and the amount of compute power you need. Their lineup includes Nvidia A100, Nvidia A40, and Nvidia T4 GPUs. Replicate’s pricing model is pay-as-you-go, billed by the second, which means you only pay for what you use.
Here’s a quick snapshot of Replicate’s GPU offerings:
- Nvidia A100 (80GB): $0.001400/sec ($5.04/hr)
- Nvidia A40 (Large) GPU: $0.000725/sec ($2.61/hr)
- Nvidia T4 GPU: $0.000225/sec ($0.81/hr)
Fal.ai’s Cloud GPU Models
Fal.ai, on the other hand, focuses on blazing-fast diffusion models and high-performance generative AI workloads. They leverage the Nvidia A100 and Nvidia A6000 GPUs. Like Replicate, Fal.ai offers a pay-for-what-you-use model, but they also provide the option to be billed by the output of generated images for specific models like Stable Diffusion 3.
Here’s a breakdown of Fal.ai’s main GPU options:
- Nvidia A100 (40GB): $0.00111/sec
- Nvidia A6000 (48GB): $0.000575/sec
- Billing per output for some models (e.g., $0.035 per Stable Diffusion image)
Pricing Breakdown
One of the most important factors in selecting a Cloud GPU provider is the cost. Here’s how Replicate and Fal.ai stack up:
Replicate Pricing
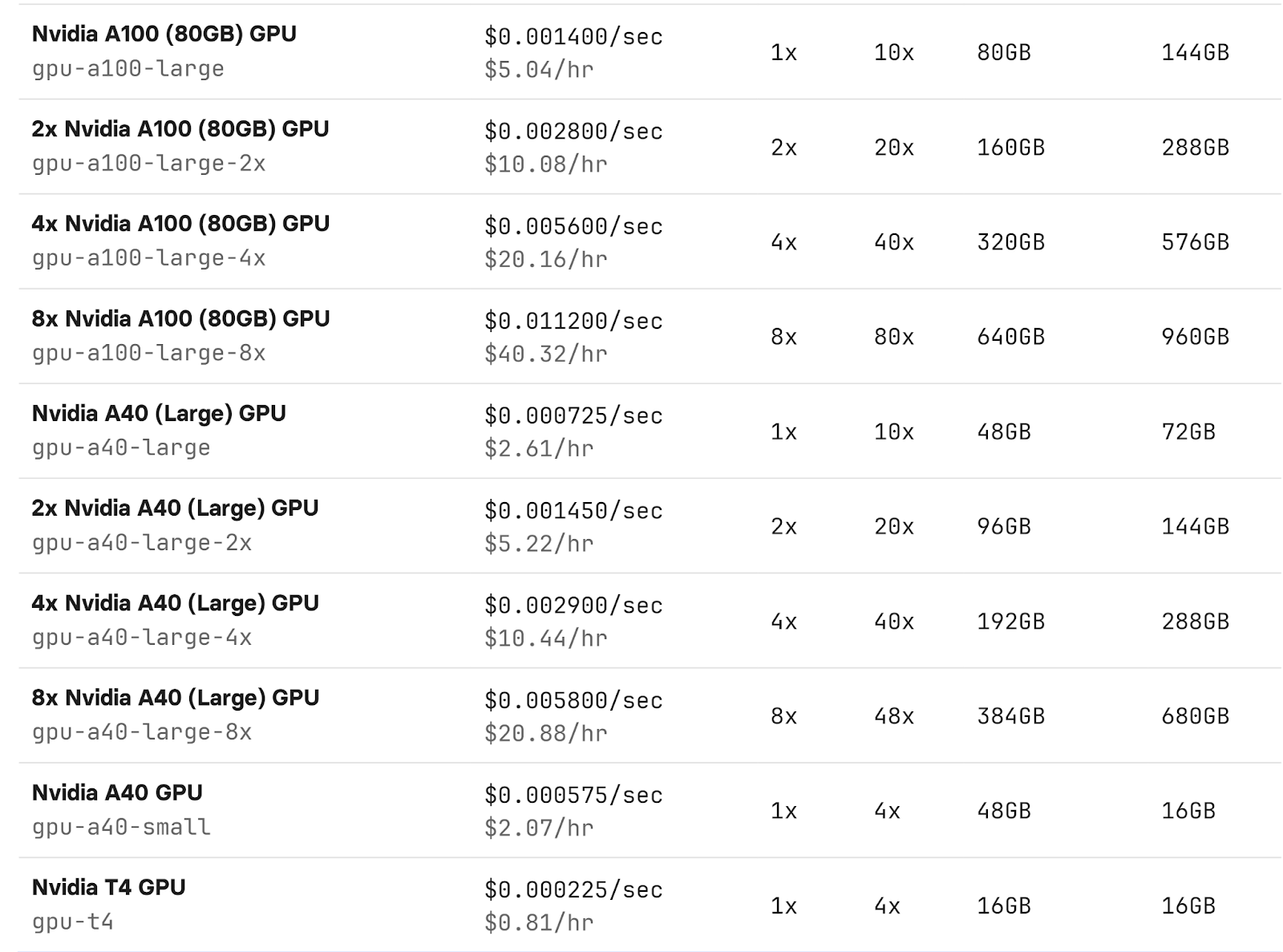
Replicate’s pricing is extremely straightforward. You’re billed per second for the time your AI models are running. If you’re not using the service, the cost automatically scales down to zero, which is ideal if you have unpredictable workloads or need cost-effective solutions.
Here are the core prices for Replicate:
- Nvidia A100 (80GB): $0.001400 per second
- Nvidia A40 (Large) GPU: $0.000725 per second
- Nvidia T4 GPU: $0.000225 per second
If you’re working with image generation tasks like Stable Diffusion or FLUX, costs can be as low as $0.03 per image for basic models, and they scale depending on your custom needs. For more advanced models like black-forest-labs/flux-pro, you might pay $0.055 per image.
Elevate your AI and machine learning projects with CUDO Compute’s robust cloud GPUs. Begin scaling today— Sign up now!
Fal.ai Pricing
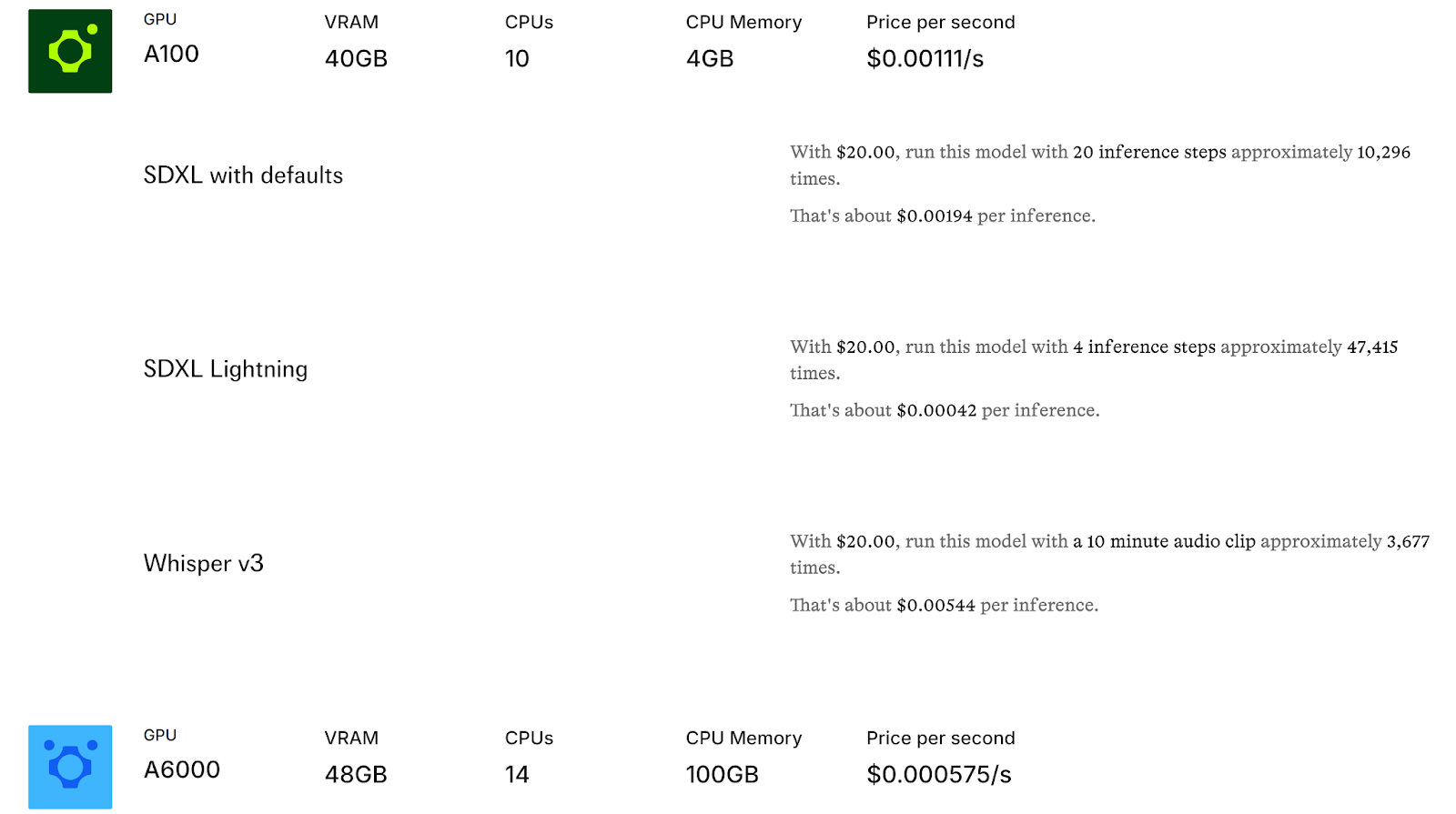
Fal.ai offers competitive pricing, particularly when it comes to the specific needs of generative AI workloads. Like Replicate, Fal.ai bills by the second, but they also offer billing based on the output of models. This approach is useful if your focus is on generating a lot of content quickly and paying per output unit.
Here’s what you can expect:
- Nvidia A100 (40GB): $0.00111 per second
- Nvidia A6000 (48GB): $0.000575 per second
- Per-image pricing for Stable Diffusion 3: $0.035 per image
Both platforms offer flexible pricing, but Fal.ai’s per-output pricing can be a real advantage for users focused on generative AI and creative tasks where output volume is key.
Performance and Speed
Replicate Performance
Replicate’s Nvidia A100 and A40 GPUs are ideal for running a wide variety of machine learning models, from image processing to language tasks. These GPUs provide massive computing power to handle large models and datasets with ease. Additionally, Replicate automatically scales to meet the demands of high-traffic situations, so you don’t have to worry about managing GPU resources manually.
The Nvidia A100 GPUs in Replicate’s lineup are particularly well-suited for AI research and large-scale model training, offering up to 80GB of GPU RAM and 144GB of total RAM on a single GPU. For smaller tasks or less demanding workflows, the Nvidia T4 is a cost-effective option that still offers solid performance at a much lower price point.
Fal.ai Performance
Fal.ai’s big selling point is its Fal Inference Engine™, which claims to be one of the fastest ways to run diffusion models. They boast speeds up to 400% faster than other platforms for running specific models like Stable Diffusion. This makes Fal.ai an attractive option if you’re looking for lightning-fast image generation or real-time AI-driven media.
For example, Fal.ai’s A100 GPUs can run Stable Diffusion at a cost as low as $0.00194 per inference, and you can run SDXL Lightning with just 4 steps for $0.00042 per inference. This makes Fal.ai particularly useful for real-time applications, like network security or generative AI tasks that require near-instant results.
Looking for flexible and scalable GPU cloud services for AI or rendering? CUDO Compute provides dependable solutions at competitive rates. Sign up now!
Use Cases for Different GPUs
Let’s look at the specific use cases for the GPUs available on both platforms.
Nvidia A100 (Replicate)
The Nvidia A100 is a beast when it comes to high-performance AI tasks. With 80GB of GPU RAM, it’s perfect for training large-scale machine learning models, running inference on complex datasets, and performing intensive tasks like fine-tuning models with custom datasets. This makes it ideal for researchers and developers working with next-generation AI models.
Some examples of where the A100 shines:
- Training deep learning models for language and image recognition.
- Running inference on stable diffusion models and other image-generating tasks.
- Scaling AI applications to handle millions of users or large datasets.
Nvidia A40 (Replicate)
The Nvidia A40 is slightly more cost-effective than the A100, offering 48GB of VRAM and performing well with most AI models. It’s a great middle-ground GPU for developers who need to balance cost and performance.
Ideal use cases include:
- Image generation tasks like FLUX or Stable Diffusion.
- Running mid-scale AI research projects.
- Fine-tuning pre-trained models with smaller datasets.
Nvidia T4 (Replicate)
If you’re looking for a budget-friendly GPU, the Nvidia T4 is an excellent choice. This versatile graphics card strikes a balance between affordability and performance, making it suitable for a variety of applications.
While the T4 may not deliver the same raw power as the higher-end A100 or A40 models, it compensates with its efficiency and capabilities tailored for specific tasks. Designed with AI workloads in mind, the T4 excels at handling lightweight AI tasks, making it a practical option for developers and organizations looking to implement machine learning solutions without breaking the bank.
One of the standout features of the T4 is its proficiency in inference for smaller machine learning models. This means it can quickly process data and generate predictions without the need for extensive computational resources. For businesses focused on real-time applications, such as chatbots or recommendation systems, the T4 can provide the necessary speed and reliability, ensuring a smooth user experience.
Moreover, the T4 is built on Nvidia’s Turing architecture, which includes dedicated Tensor Cores. These cores enhance the GPU’s ability to perform deep learning tasks, enabling efficient processing of neural networks. This makes the T4 particularly well-suited for training smaller models and running inference tasks that require quick response times.
In addition to its performance capabilities, the T4’s low power consumption is a significant advantage. With a thermal design power (TDP) of just 70 watts, it is energy-efficient, helping to keep operational costs down. This efficiency, coupled with its price point, makes the T4 a smart investment for startups or projects with budget constraints.
Use Cases for the Nvidia T4
- Real-Time Inference: Ideal for applications like chatbots and virtual assistants that require quick, responsive interactions.
- Recommendation Systems: Effective for processing user data to generate personalized content suggestions in e-commerce or streaming platforms.
- Image and Video Analysis: Suitable for lightweight tasks such as object detection and facial recognition in surveillance systems.
- Natural Language Processing (NLP): Great for smaller NLP models, enabling text classification and sentiment analysis without heavy resource demands.
- Data Analytics: Efficient for executing machine learning algorithms on structured data, helping businesses derive insights from their datasets.
- Edge Computing: Perfect for deployment in edge devices that require low power usage but still need capable AI processing.
Gain immediate access to NVIDIA and AMD GPUs on-demand with CUDO Compute—ideal for AI, ML, and HPC projects. Sign up now!
Nvidia A6000 (Fal.ai)
Fal.ai’s Nvidia A6000 provides 48GB of VRAM, and like Replicate’s A40, it’s a great option for cost-effective AI workloads. However, the A6000 is particularly well-optimized for generative AI tasks, thanks to Fal.ai’s custom Inference Engine.
Some of the best use cases include:
- Running generative media tasks with high throughput.
- Deploying custom docker image containers to run inference.
- Scaling generative AI workloads for real-time use cases like network security or creative applications.
Deployment Process and Ease of Use
Replicate’s Deployment Process
Replicate offers a straightforward deployment process. You can get started with just a few lines of code, thanks to their robust API. Replicate allows you to deploy custom AI models or use thousands of pre-built models contributed by the community.
Deploying your models on Replicate is as simple as running the following command through their platform. For more advanced deployments, you can use their Docker container system to package your models and run them at scale.
Fal.ai’s Deployment Process
Fal.ai makes deployment similarly easy. With their Fal API, you can deploy your models efficiently and even fine-tune your own diffusion models. Similar to Replicate, Fal.ai also supports Docker images, granting users the flexibility to manage and scale their AI workloads effectively.
This compatibility with Docker means you can easily deploy applications in a consistent environment, reducing the chances of unexpected issues during scaling. Whether you’re deploying a simple model or a more complex setup, Fal.ai’s deployment process is designed to minimize friction and maximize productivity.
Scalability and Flexibility
Scalability and flexibility are essential features for any cloud service, especially when it comes to GPU models. Both Replicate and Fal.ai shine in this area, ensuring your applications can grow and adapt without a hitch.
Replicate takes scalability to the next level by automatically scaling GPU resources based on your demands. This means that during peak usage times, it ramps up the power you need, allowing for smooth performance even under heavy loads. When demand decreases, Replicate efficiently scales down to zero, helping you save on costs without compromising on performance. This on-demand model ensures you’re only paying for what you use, making it a budget-friendly option.
Fal.ai, on the other hand, excels in handling unpredictable traffic patterns. Its real-time infrastructure allows it to adjust dynamically, seamlessly managing spikes in user activity. Whether you’re launching a new feature that attracts sudden interest or facing a surge in user requests, Fal.ai responds swiftly, ensuring that your application remains responsive. This adaptability not only enhances user experience but also provides peace of mind, knowing your infrastructure can handle whatever comes its way.
Both platforms offer robust solutions for scalability and flexibility, but they cater to different needs. Replicate is ideal for those looking for straightforward scaling based on usage, while Fal.ai is perfect for applications requiring real-time adjustments to fluctuating traffic demands.
Replicate vs Fal.ai: Conclusion
Replicate’s wide range of GPUs, strong community support, and pay-for-what-you-use model make it a great choice for those looking to deploy or fine-tune machine learning models with flexibility and ease. Its scaling capabilities and support for custom Docker containers give developers the tools they need to handle complex AI workloads without worrying about infrastructure management.
On the other hand, Fal.ai stands out for its blazing-fast inference engine, specifically optimized for generative AI and image generation tasks. If you’re focused on stable diffusion or real-time creative applications, Fal.ai’s ability to offer cost-effective solutions with per-output billing may be a game-changer. With support for running diffusion models up to 4x faster than other platforms, it’s ideal for those who prioritize speed and efficiency in their AI deployments.
So, which one should you choose?
- If you’re working on diverse AI projects and need flexibility in choosing between GPU models, Replicate offers a more general approach with a wide variety of pricing options and AI research support.
- If your focus is heavily on creative AI tasks like generating images or running high-speed diffusion models, Fal.ai’s performance-optimized infrastructure might be the better fit, especially for those who want scalable solutions that are built for next-generation AI applications.
In the end, both platforms have their own strengths. Your decision should be based on the specific needs of your project, whether it’s about maximizing computing power, optimizing costs, or running new models quickly and efficiently.
CUDO Compute provides high-performance, budget-friendly cloud GPU solutions tailored to your AI and ML needs. Sign up now!