Lambda Labs Review: Best Cloud Provider for AI Workloads?
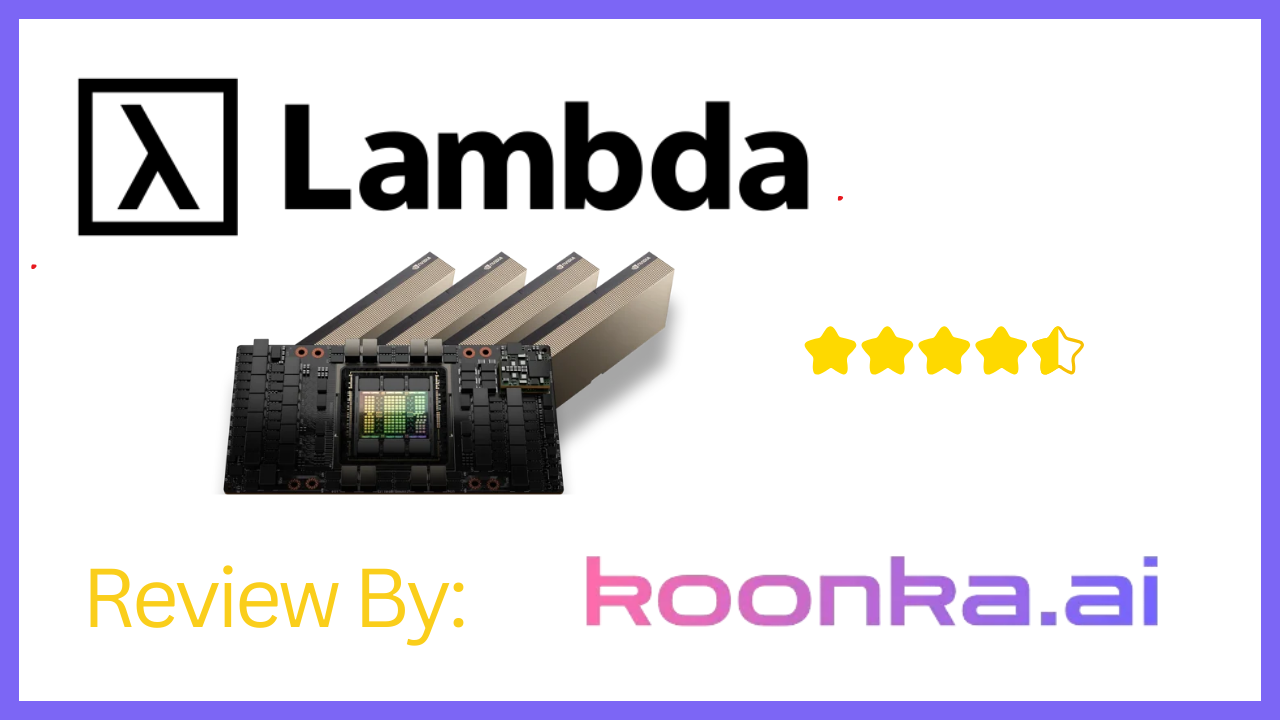
When it comes to deep learning, AI training, and large-scale model deployment, finding a reliable, powerful, and cost-efficient cloud GPU provider can make or break your projects. Lambda Labs is a key player in this space, offering specialized cloud services for machine learning workloads. The platform promises top-tier GPUs, transparent pricing, and an easy-to-use interface for developers and researchers. But does it deliver on all fronts?
In this Lambda Labs review, we’ll dive into everything Lambda Labs has to offer, covering GPU options, pricing, infrastructure, ease of use, customer support, and much more. Whether you’re looking to spin up a single instance or scale across thousands of GPUs, you’ll get a comprehensive look at whether Lambda Labs is the right cloud solution for your needs.
Affiliate Disclosure
We are committed to being transparent with our audience. When you make a purchase via our affiliate links, we may receive a commission at no extra cost to you. These commissions support our ability to deliver independent and high-quality content. We only endorse products and services that we have personally used or carefully researched, ensuring they provide real value to our readers.
Table of Contents
Lambda Labs Review: Our Experience
We found Lambda Labs GPU Cloud to be a decent solution for AI professionals. Its range of powerful GPUs, seamless setup, and strong AI-centric design make it a solid option for model training and inference. However, it may not be the best choice for every use case due to certain limitations.
Looking for affordable, high-performance GPUs? With CUDO Compute, you can easily access top-tier NVIDIA and AMD GPUs whenever you require them. Sign up now!
To learn more about CUDO Compute, take a look at this video:
Lambda Labs Cloud: Pros and Cons
Pros:
- Wide range of NVIDIA GPUs: Access to H100, A100, and A10 GPUs, and more, for different AI workload requirements.
- Transparent pricing: Pay for only what you use with no hidden costs like egress fees.
- Pre-configured ML environments: Comes with Ubuntu, TensorFlow, PyTorch, CUDA, and other essential ML frameworks pre-installed.
- Developer-friendly API: Easy to automate instance management with a simple API.
- Fast networking speeds: Quantum-2 InfiniBand with 3200 Gbps bandwidth optimized for distributed training.
- No long-term contracts: Spin up instances when needed without commitment.
Cons:
- Limited global availability: Instance availability is restricted to select regions like the USA, EU, and Japan.
- No GUI support: Designed for command-line use, making it less suitable for rendering tasks or users needing graphical interfaces.
- No backup once instance is terminated: You must ensure your data is backed up before the instance shutdown.
- High pricing for top-tier GPUs: H100 and A100 instances can be expensive compared to other cloud GPU providers.
- Additional storage cost: Persistent storage is an additional cost.
- Limited payment options: Debit and prepaid cards are not accepted, limiting payment options.
- Poor customer support: You’ll get slow responses without any 24/7 live support.
GPU Models and Pricing
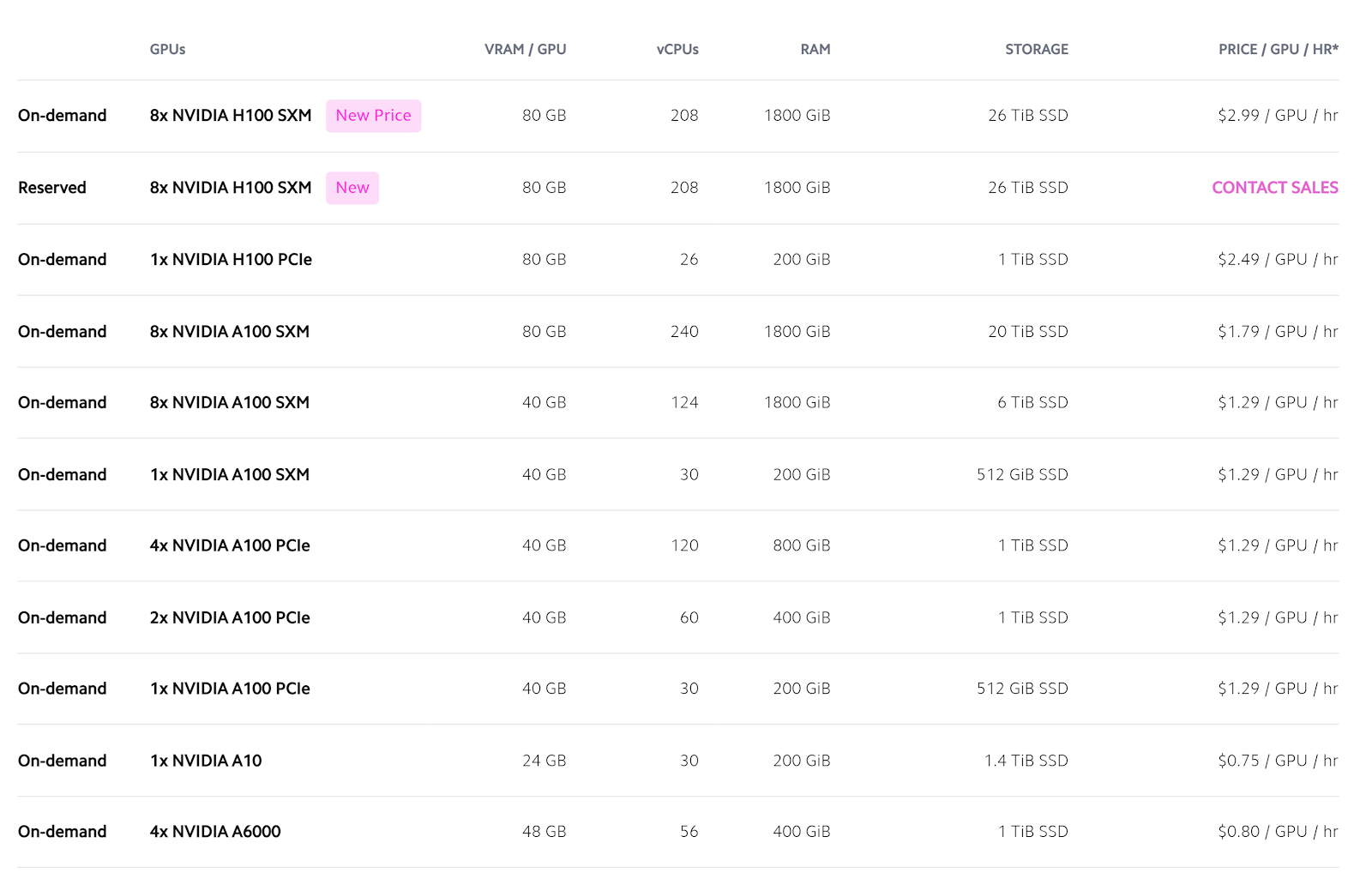
Lambda Labs offers a wide range of GPUs that cater to different workloads and budgets. Below is an overview of the key GPU models, their specs, and on-demand pricing:
NVIDIA H100 Tensor Core (80 GB VRAM)
Pricing: $2.49 – $2.99 per GPU/hour
Ideal for: Large-scale AI models, foundation models, LLMs (Large Language Models), generative AI, and high-performance computing.
Features and Performance: The NVIDIA H100 Tensor Core GPU is a beast when it comes to handling massive workloads, especially in the realms of AI and deep learning. It comes equipped with 80 GB of HBM3 memory (High Bandwidth Memory), which provides an impressive 3.35 TB/s of bandwidth, making it perfect for data-intensive tasks. This GPU is designed for speed and efficiency, handling everything from large neural networks to high-throughput inference tasks with ease.
The H100 also includes 4th Gen Intel Xeon processors and the Transformer Engine, optimized for FP8 precision, offering significant performance boosts in AI model training. The second-generation Multi-Instance GPU (MIG) technology enables the H100 to partition itself into multiple smaller GPU instances, maximizing flexibility and utilization for different workflows. It also integrates with NVLink and NVSwitch interconnects, ensuring fast communication between GPUs, crucial for distributed model training.
Use Cases:
- Training and fine-tuning large AI models like GPT, BERT, and DALL-E.
- Handling Generative AI tasks, such as producing complex content from large datasets.
- Suitable for organizations working on long-term research projects and developers handling cutting-edge AI innovations.
- Ideal for large-scale inference tasks, where data throughput is critical.
The H100 is one of the latest additions to the Lambda Cloud and is a go-to option for high-end AI developers and machine learning engineers aiming for top-tier performance.
NVIDIA A100 Tensor Core (80 GB and 40 GB VRAM)
Pricing: $1.29 – $1.79 per GPU/hour
Ideal for: AI training, fine-tuning models, and large-scale deep learning tasks.
Features and Performance: The NVIDIA A100 Tensor Core GPU is a versatile and highly sought-after GPU model, known for its flexibility across different machine learning workloads. Available in both 80 GB and 40 GB configurations, the A100 excels in parallel computing, thanks to its multi-instance GPU (MIG) technology, which allows a single GPU to be divided into multiple instances, each handling its own workload.
With HBM2e memory and up to 1.6 TB/s of memory bandwidth, the A100 offers balanced performance for both training and inference tasks. For users requiring flexibility, the A100 is configurable with up to 240 vCPUs and 1800 GiB of RAM, making it suitable for a wide range of machine learning applications.
Use Cases:
- Ideal for both training and fine-tuning AI models such as image recognition systems and natural language processing (NLP) models.
- Suitable for AI researchers or data scientists building neural networks from scratch or fine-tuning pre-trained models.
- Can handle real-time inference at scale, making it an excellent choice for AI deployment in production environments.
- Well-suited for multi-GPU distributed training, where flexibility and cost control are key.
For developers looking for an all-rounder GPU that delivers top-tier performance without the cost of the H100, the A100 is an excellent choice, blending high computing power with affordability.
Boost your AI and machine learning projects with the robust cloud GPUs provided by CUDO Compute — Sign up now!
NVIDIA A10 and A6000
Pricing: $0.75 – $0.80 per GPU/hour
Ideal for: Inference tasks, smaller models, budget-conscious developers, and lighter workloads.
Features and Performance: The NVIDIA A10 and A6000 GPUs are solid options for developers looking to perform cost-effective deep learning tasks. The A10 offers 24 GB of VRAM, while the A6000 provides 48 GB, giving users plenty of memory for lighter machine-learning models and inference tasks. Both models use GDDR6 memory, which provides fast data access, although they don’t match the bandwidth of the more advanced H100 or A100 models.
The A10 and A6000 are particularly suitable for inference, meaning they excel at taking pre-trained models and generating outputs, rather than training models from scratch. These GPUs are optimized for graphics-intensive tasks, like rendering and visualization, as well as basic AI workloads. They are also significantly more affordable than high-end GPUs, making them an attractive choice for startups or developers working with smaller datasets or less compute-heavy models.
Use Cases:
- Best suited for cost-sensitive projects that don’t require the extensive computational power of the H100 or A100.
- Ideal for developers working on small to medium-sized ML models that are computationally light, such as recommendation systems or classification algorithms.
- Excellent for real-time inference in production, especially for AI applications requiring quick response times with smaller datasets.
- Also suitable for tasks like 3D rendering and computer-aided design (CAD), thanks to their graphical processing capabilities.
While the A10 and A6000 are not as powerful as the H100 or A100, they still pack a punch for users who need reliable performance at an affordable price.
NVIDIA Tesla V100
Pricing: $0.55 per GPU/hour
Ideal for: Users needing a balance between price and performance for mid-range AI workloads.
Features and Performance: The NVIDIA Tesla V100 is an older generation GPU but remains a reliable option for users who need strong performance without the premium pricing of newer models. Equipped with 16 GB of HBM2 memory and 900 GB/s bandwidth, the V100 is well-suited for a wide range of AI tasks, including deep learning, machine learning, and data analytics.
Although it’s not as powerful as the H100 or A100 models, the V100 still supports Tensor Cores for accelerating mixed-precision training, which makes it a great option for both training and inference, especially for mid-sized models. The Volta architecture ensures high computational efficiency, making it suitable for users who don’t need cutting-edge performance but still require a strong workhorse GPU.
Use Cases:
- A great fit for mid-range deep learning tasks, such as training convolutional neural networks (CNNs) or recurrent neural networks (RNNs).
- Ideal for developers or researchers working on proof-of-concept models or initial experimentation, where cost-effectiveness is a key factor.
- Can handle real-time inference for smaller models, though it may struggle with the demands of very large datasets or models.
- Suitable for data analytics tasks and scientific computing where high memory bandwidth is necessary but extreme computational power isn’t required.
For users who don’t need the latest hardware and are more focused on keeping costs low, the Tesla V100 is a tried-and-true option that still holds up well in 2024.
These GPU models cater to a wide variety of workloads, from massive AI projects to smaller, cost-conscious development tasks. Whether you need cutting-edge performance or an affordable solution for machine learning, Lambda Labs has a GPU option that fits the bill.
Looking for adaptable and scalable GPU cloud services for AI or rendering? CUDO Compute provides reliable solutions at competitive rates. Sign up now!
Infrastructure: Speed and Performance
Lambda Labs doesn’t just rely on powerful GPUs; it has built an infrastructure optimized for AI workloads. Here’s a closer look:
GPU Instances
The platform supports multi-GPU configurations (up to 8 GPUs per instance) and is designed for scalability. Whether you’re training models on a single H100 GPU or across 8 GPUs, Lambda’s infrastructure ensures minimal latency and high-speed execution.
Networking
Lambda Labs uses NVIDIA Quantum-2 InfiniBand networking, delivering up to 3200 Gbps bandwidth per node. This is ideal for distributed training tasks that involve massive datasets and large models, such as training large language models (LLMs) or running generative AI workflows. Additionally, the use of GPUDirect RDMA (Remote Direct Memory Access) allows GPU-to-GPU communication without involving the CPU, reducing latency significantly.
Storage
Persistent storage on Lambda Labs is another notable feature, though it comes at an additional cost. Storage is billed per GB used per month in one-hour increments, which is fair but can add up if you’re working with large datasets.
However, Lambda’s storage solution lacks flexibility in terms of backup and recovery. If you terminate an instance without backing up your data, it’s gone forever. This makes the use of external storage solutions or regular backups essential.
Ease of Use
Lambda Labs markets itself as a developer-friendly platform, and it generally lives up to that promise.
Lambda Stack
All instances come pre-installed with a comprehensive software stack (TensorFlow, PyTorch, CUDA, etc.), so you can start training your models almost immediately on this deep-learning workstation. This is particularly useful for researchers who want to avoid the hassle of setting up environments and installing dependencies.
Jupyter Notebooks
Lambda offers one-click Jupyter access, allowing you to run notebooks directly from your browser. This is a convenient feature for ML engineers and data scientists who prefer a notebook-based workflow.
API Access
For those looking to automate workflows, Lambda Labs provides a Cloud API. You can launch, terminate, and restart instances programmatically, making it easier to integrate with other systems or scale efficiently.
However, ease of use has its limits. For instance, there’s no support for a typical desktop interface—everything is done via SSH or Jupyter. This can be a drawback for users unfamiliar with command-line interfaces.
CUDO Compute offers instant access to NVIDIA and AMD GPUs on demand, perfect for AI, machine learning, and high-performance computing requirements. Sign up now!
Network and Speed
Networking speed is one of Lambda’s strongest features. With the NVIDIA Quantum-2 InfiniBand networking, you get access to the absolute fastest network available for distributed training tasks.
GPUDirect RDMA
This feature allows for direct communication between GPUs, skipping the CPU entirely and drastically reducing communication latency. For large-scale distributed training, this is a game-changer, especially when dealing with thousands of GPUs.
Non-blocking Network Topology
Lambda employs a non-blocking multi-layer topology with zero oversubscription, ensuring that each GPU in the cluster has full access to the network’s bandwidth. This is critical when scaling across multiple GPUs, as it avoids bottlenecks that can slow down training.
Customer Support and Documentation
Lambda Labs provides a decent support system, but it’s not without flaws.
Support Quality
Lambda offers both standard and premium support options. Premium support includes assistance with issues related to PyTorch, TensorFlow, and CUDA. However, most of the users have reported inconsistent response times, especially for urgent issues. The platform does not offer 24/7 live support, which could be a deal-breaker for enterprises relying on round-the-clock services.
Documentation
The documentation available on the Lambda website is comprehensive and includes everything from instance setup to API usage. It covers the most common queries and provides step-by-step instructions for beginners, especially useful for users new to SSH keys or cloud computing.
CUDO Compute offers powerful and affordable cloud GPU solutions specifically designed for your AI and machine learning requirements. Sign up now!
Regions and Availability
Lambda Labs is not globally available, which can be a significant limitation for users outside the supported regions. As of now, the service is available in the USA, Canada, Chile, the EU (excluding Romania), Switzerland, the UK, UAE, South Africa, Israel, Taiwan, Japan, Korea, Singapore, Australia, and New Zealand.
If you’re based in a region not supported by Lambda Labs, you’ll have to look elsewhere for cloud GPU services. This limitation is particularly frustrating for developers in regions with high demand for GPU computing, like India or Southeast Asia.
Billing and Payments
Lambda Labs uses a straightforward billing system where you are charged per minute of GPU usage. This flexibility allows for granular control of costs, making it easier to budget for projects. However, there are some limitations:
- No Debit/Prepaid Cards: Lambda does not accept debit or prepaid cards, which may limit access for smaller teams or individuals.
- Sales Tax: As of 2024, Lambda charges sales tax based on your billing address, so keep that in mind when calculating your total costs.
Refunds are given as credits toward future usage and cannot be redeemed for cash. This is not ideal for everyone but works for long-term users who plan to stick with Lambda Labs.
Lambda Labs Review: Our Conclusion
Lambda Labs is a solid choice for anyone needing access to high-performance GPUs for AI and machine learning workloads. With top-tier GPUs like the H100 and A100, an optimized infrastructure for distributed training, and a transparent pricing model, it’s a strong contender in the cloud GPU market.
However, it’s not without its drawbacks. The platform could improve its customer support and regional availability, and the lack of GUI support limits its use cases to primarily AI-related tasks. If these limitations are deal-breakers, you may want to consider other providers, but for AI researchers and ML engineers, Lambda Cloud remains a strong option.
Lambda Labs Cloud: Frequently Asked Questions
Who are Lambda Labs competitors?
Lambda Labs competes with several cloud GPU providers, including CUDO Compute, AWS, Google Cloud, Azure, and Paperspace. These competitors offer similar GPU instances for AI and machine learning workloads but with broader services, better global coverage, and more robust ecosystem integrations. Lambda Labs stands out for AI-specific optimization, though it may lack the flexibility of larger platforms.
Is Lambda Labs worth it?
Lambda Labs can be worth it if you need powerful GPUs for AI workloads, but it’s not for everyone. While it offers strong hardware like the NVIDIA H100 and flexible pricing, its lack of broader support for other cloud services and limitations in geographic availability might not suit all users. It’s ideal for ML-heavy tasks but could feel restrictive for general cloud needs.
Who uses Lambda Labs?
Lambda Labs is widely used by AI researchers, data scientists, machine learning engineers, and enterprises working on AI, deep learning, and large-scale computational tasks. Startups, academic institutions, and established companies in industries like healthcare, automotive, and finance also leverage Lambda’s GPUs for their machine learning and AI workloads.
CUDO Compute offers high-performance and cost-effective cloud GPU solutions tailored to meet the needs of your AI and machine learning projects. Sign up now!