Lambda Labs vs Google Colab: The Best Cloud GPU Service for Your AI Projects?
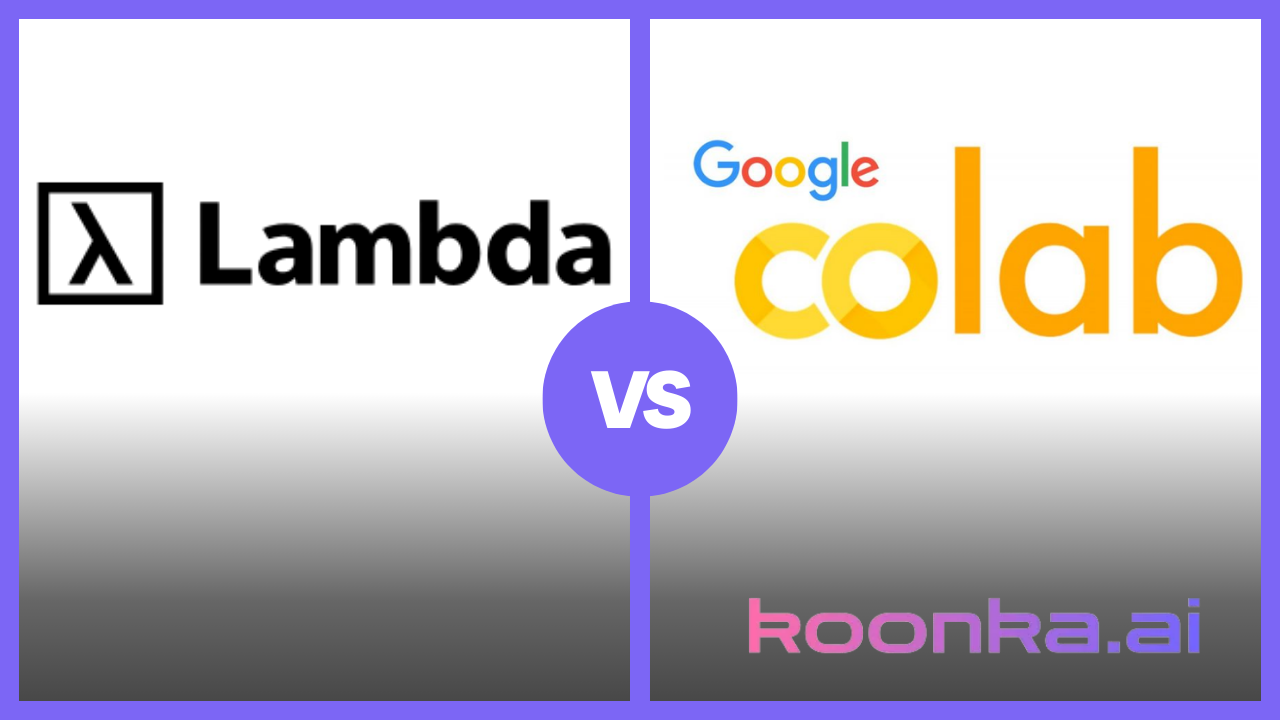
Looking to supercharge your AI projects with cloud GPU compute? Lambda Labs and Google Colab both offer GPU services, but which one fits your needs better? Lambda Labs provides access to high-performance NVIDIA H100s and H200s, perfect for intense AI training. Meanwhile, Google Colab is a favorite among developers for its free tier and ease of use.
But when it comes to GPU models and pricing, how do these two services really compare? In this Lambda Labs vs Google Colab comparison, we’ll dive into the specifics of what each platform offers and help you figure out which one might be the better choice for your AI workloads. Stick around as we explore the details.
Table of Contents
Lambda Labs vs Google Colab: The Key Difference
The main difference is that Lambda Labs offers cutting-edge, high-performance NVIDIA GPUs like H100 and A100 for demanding AI workloads, while Google Colab provides more affordable, older GPU options, making it better suited for smaller-scale machine learning projects and individual developers.
Affiliate Disclosure
We prioritize transparency with our readers. If you purchase through our affiliate links, we may earn a commission at no additional cost to you. These commissions enable us to provide independent, high-quality content to our readers. We only recommend products and services that we personally use or have thoroughly researched and believe will add value to our audience.
GPU Compute Comparison
When it comes to choosing a service for GPU compute, the hardware you get access to is key. Both Lambda Labs and Google Colab offer some powerful GPU options, but the differences lie in the variety, pricing, and suitability for various machine learning tasks.
If you need powerful GPUs without breaking the bank, CUDO Compute lets you rent top-tier NVIDIA and AMD graphics cards on demand. Sign up now!
To find out more about CUDO Compute, please watch the video below:
Lambda Labs: Cutting-Edge NVIDIA GPUs
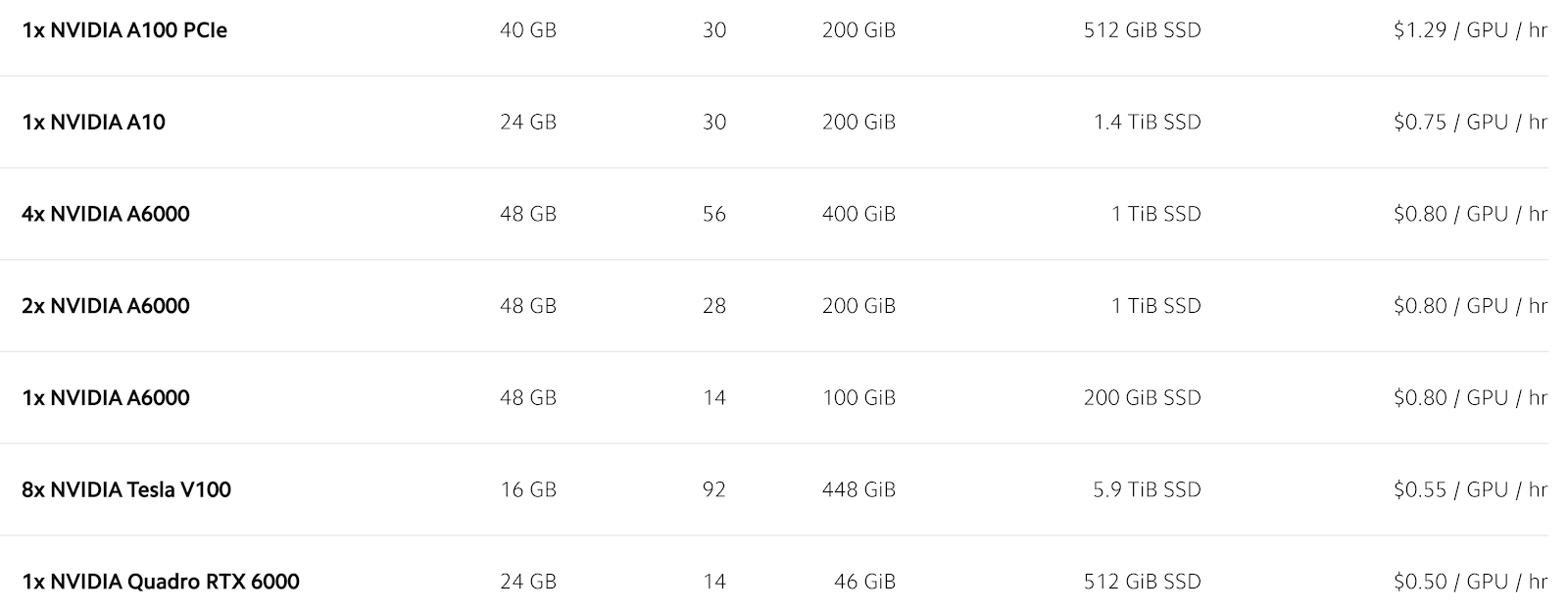
Lambda Labs is all about flexibility and high-performance compute. Their on-demand cloud provides access to NVIDIA’s most powerful GPUs, including the H100 Tensor Core and A100 models. Here’s a detailed look at the available models and their pricing:
- NVIDIA H100 Tensor Core (On-Demand)
- Price: $2.49 – $4.49 per GPU/hour
- Specs: 80 GB of HBM3 memory with a bandwidth of 3.35TB/s
- Use Cases: Perfect for training and fine-tuning large language models (LLMs), transformer-based models, and AI applications requiring heavy compute loads.
- NVIDIA H200 Tensor Core
- Price: Contact for pricing (available for extended reservations)
- Specs: 141GB of HBM3e memory at 4.8TB/s
- Use Cases: Ideal for even more demanding AI workloads, with double the memory and 1.4x the bandwidth of the H100. This model is a go-to for large-scale generative AI projects and cutting-edge LLMs.
- NVIDIA B200 Tensor Core
- Price: Contact for pricing
- Specs: 180GB of HBM3e memory with 8TB/s bandwidth
- Use Cases: Optimized for training and inference in large models, the B200 represents the latest in NVIDIA’s Blackwell architecture, designed for highly demanding distributed AI workloads.
- NVIDIA A100 Tensor Core
- Price: $1.29 – $1.79 per GPU/hour
- Specs: 40 GB or 80 GB versions, supporting a wide range of compute instances
- Use Cases: Suitable for tasks ranging from LLM training to running custom machine learning models. This GPU is versatile enough for deep learning, AI research, and large-scale inference.
- NVIDIA A6000
- Price: $0.80 per GPU/hour
- Specs: 48 GB of GDDR6 memory
- Use Cases: Great for mid-tier AI projects and training that still require high memory bandwidth but don’t need the compute muscle of the H100 or A100.
- NVIDIA V100 Tensor Core
- Price: $0.55 per GPU/hour
- Specs: 16 GB of memory
- Use Cases: Best suited for less memory-intensive machine learning tasks and older models. Still powerful, but not on the same level as the A100 or H100.
Lambda Labs makes it easy to scale up your compute needs with up to 512 GPUs in a single cluster. The pricing also offers flexibility depending on your time commitment. The on-demand prices might seem high, but the discounts for long-term reservations can save you up to 45%.
Enhance your AI and machine learning projects using CUDO Compute’s powerful cloud GPUs. Start scaling your initiatives now— Sign up now!
Google Colab: Affordable GPU Options
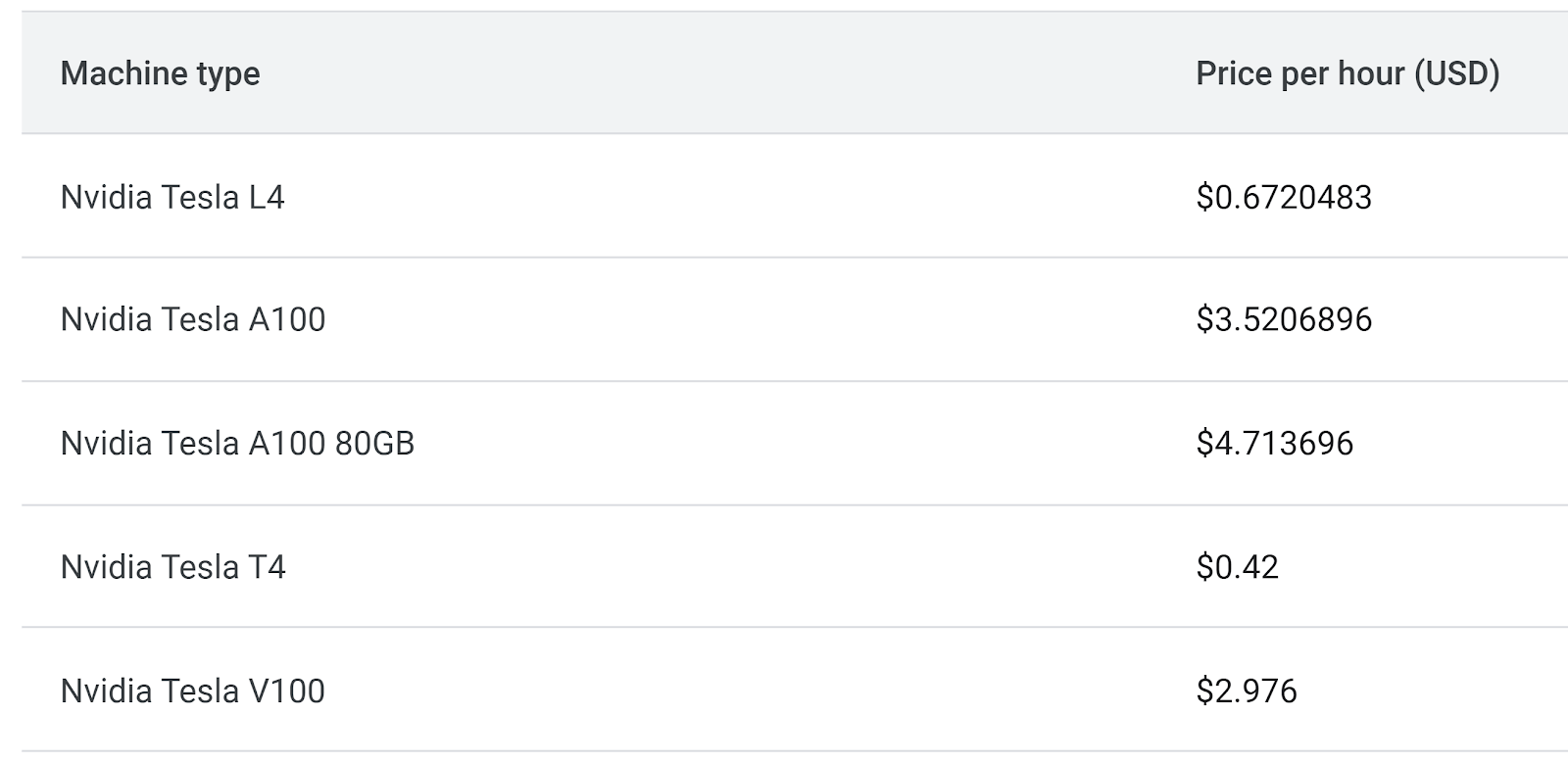
Google Colab, on the other hand, offers a more budget-friendly option, especially for individual developers and smaller teams. The GPU models available through Colab are slightly older, but still powerful enough for many machine learning tasks. Here’s the rundown:
Google Colab offers different GPU models based on the selected plan (Pro, Pro+, or Enterprise):
- Nvidia Tesla L4:
- Price per hour: $0.67
- Use Case: Suitable for small- to medium-sized machine learning projects that don’t require extensive computational power. It works well for fine-tuning models, interactive voice response system testing, and other lightweight AI tasks.
- Nvidia Tesla T4:
- Price per hour: $0.42
- Specs: 16 GB GDDR6 memory
- Use Case: A balanced GPU for training custom image models, providing adequate power for training natural language understanding platforms and executing machine learning models in a relatively cost-effective manner.
- Nvidia Tesla V100:
- Price per hour: $2.98
- Specs: 32 GB HBM2 memory
- Use Case: This GPU is ideal for more intensive workloads, such as data matching tools, training large language models, or building dynamic and targeted recommendations for users.
- Nvidia Tesla A100:
- Price per hour: $3.52
- Specs: 40 GB or 80 GB memory options available
- Use Case: Perfect for large-scale AI training, including custom machine learning models. It’s often used in sophisticated collective intelligence networks, such as those predicting global consumer preferences.
- Nvidia Tesla A100 80GB:
- Price per hour: $4.71
- Specs: 80 GB memory
- Use Case: Built for massive data workloads, including real-time data processing and intensive AI model training. The extended memory helps with large datasets, improving efficiency and reducing training times.
Looking for adaptable and scalable GPU cloud services for AI or rendering? CUDO Compute provides reliable solutions at affordable rates. Sign up now!
Performance and Scaling
Performance is a crucial factor when it comes to GPU compute. Whether you’re working on training large language models, running inference for dynamic recommendations, or testing out industry-leading prediction accuracy algorithms, performance and scalability can make or break a project.
Let’s look at how Lambda Labs and Google Colab compare in terms of scaling up workloads and handling demanding tasks.
Multi-GPU Support
Lambda Labs:
Lambda Labs offers multi-GPU instances, meaning you can scale your workloads efficiently across several GPUs, with options like 1x, 2x, 4x, and 8x NVIDIA GPUs. For example, Lambda’s H100 and A100 instances allow scaling up to 512 GPUs, making it perfect for distributed training across multiple nodes.
This is particularly beneficial for workloads like sophisticated collective intelligence networks or natural language understanding platforms, where large datasets need to be processed in parallel.
The ability to spin up multi-GPU clusters also provides more flexibility in pricing. For example, if you reserve a cluster of H100 GPUs for 12 months, the hourly price can drop as low as $2.49 per GPU, providing significant savings for long-term projects.
Google Colab:
Colab, on the other hand, is much more limited when it comes to multi-GPU support. While it offers access to powerful GPUs like the Tesla V100, it doesn’t allow for clusters of GPUs in the same way Lambda Labs does.
Colab is designed more for single-instance use, making it a better fit for small-scale, individual projects rather than enterprise-level training of large models.
However, to execute machine learning models or for training models for virtual agent services, Colab’s performance is more than enough.
With CUDO Compute, you can instantly access NVIDIA and AMD GPUs on-demand, making it perfect for AI, machine learning, and high-performance computing projects. Sign up now!
Auto-Scaling
Lambda Labs:
Lambda Labs doesn’t just support large-scale GPU usage—it’s also designed to scale dynamically. You can easily expand your GPU cluster as your compute needs grow. Whether you’re training a large foundation model like LLM360 or deploying inference models for real-time applications, Lambda’s auto-scaling options ensure that you don’t need to worry about hitting performance bottlenecks.
Lambda’s cloud is optimized for distributed workloads, meaning you can run large models across thousands of GPUs simultaneously without hitting network bottlenecks, thanks to their non-blocking InfiniBand networking. This allows you to maintain full bandwidth across your entire setup, ensuring smooth operation even for massive models like Falcon or Mistral.
Google Colab:
Google Colab doesn’t have the same level of auto-scaling as Lambda Labs. Instead, it provides different compute units, which users can purchase as needed. This is more suitable for smaller projects or developers who want to run short-term experiments.
For example, if you’re developing a data matching tool or testing out Google Cloud’s Vision API, Colab’s pay-as-you-go model might be sufficient.
Colab’s flexible pay-as-you-go pricing is a great option for users who don’t require constant access to large clusters of GPUs but need something for short bursts of work.
GPU Networking and Data Transfer
Networking can often be an overlooked aspect of GPU compute, but it’s absolutely essential for those working with large datasets, especially in machine learning and AI projects. Efficient data transfer between nodes ensures that your GPUs are working at full capacity without being bottlenecked by slow communication speeds.
Networking in Lambda Labs
Lambda Labs boasts some of the best networking options available for GPU compute, particularly for large-scale distributed training tasks. Their infrastructure is powered by NVIDIA Quantum-2 InfiniBand, which delivers up to 3200 Gbps of bandwidth for each 8x H100 or H200 GPU cluster.
This is especially useful for multi-node setups, as it allows for direct communication between GPUs without having to go through the CPU, thanks to GPUDirect RDMA.
This infrastructure makes Lambda ideal for users working on large-scale foundation models or a natural language understanding platform. For instance, if you’re training an AI model to predict global consumer preferences or running a custom machine learning model, having such high-speed networking ensures minimal latency and maximum performance.
Networking in Google Colab
Google Colab does not provide the same level of networking performance as Lambda Labs, primarily because Colab is not built for distributed GPU clusters. Colab’s focus is more on ease of use and access, which is reflected in its reliance on Google Cloud Platform’s standard network.
This works well enough for smaller-scale applications but can become a bottleneck when dealing with larger models or distributed workloads.
That said, Colab integrates well with other Google Cloud Platform services, such as Vertex Data Labeling and BigQuery, which allows you to process and analyze large datasets more efficiently. However, if networking speed is a critical factor in your decision, Lambda Labs holds a significant edge.
CUDO Compute offers strong and cost-effective cloud GPU solutions designed specifically for your AI and machine learning requirements. Sign up now!
Ease of Use
Another key factor when choosing between Lambda Labs and Google Colab is ease of use, especially for developers who may not be as familiar with the intricacies of cloud infrastructure. Let’s break down the usability of both platforms.
Lambda Labs
Lambda Labs is designed for experienced users who are familiar with AI workflows and cloud infrastructure. The platform offers a powerful API that allows you to launch, terminate, and restart GPU instances quickly and easily. There’s also an option to manage everything through a user-friendly interface, but the API gives more control for advanced users.
One of the standout features of Lambda Labs is the Lambda Stack, which comes pre-configured with popular machine learning frameworks like TensorFlow, PyTorch, and Keras. This is especially useful for data scientists who want to hit the ground running without worrying about software setup. The platform also provides Jupyter Notebooks, enabling users to interact directly with their data in a web browser.
Lambda Labs may not be as beginner-friendly as Google Colab, but for users who need enterprise-grade tools for large-scale model training and inference, it’s an excellent option.
Google Colab
Google Colab is the go-to platform for ease of use, especially for beginners or users who don’t need massive compute power. Colab offers a simple interface where you can create and run Jupyter Notebooks directly in the cloud, without any setup. It also integrates seamlessly with Google Drive, allowing for easy file storage and sharing.
One major advantage of Google Colab is its integration with other Google services like Google Cloud’s Vision API, BigQuery, and Google Cloud Platform. This makes it an attractive option for users working with Google’s ecosystem. Plus, with features like code completion powered by generative AI and real-time collaboration, Colab offers a lot of flexibility and convenience for solo developers and small teams.
For users focused on smaller-scale tasks like building an interactive voice response system or experimenting with virtual agent services, Colab is perfect. However, for more advanced users looking to train large-scale models, Lambda Labs is likely the better choice.
Customization and Control
When it comes to customization, both platforms allow users to tailor their setups to their specific needs, but the level of control varies.
Lambda Labs:
Lambda Labs gives users a lot of control over their environment. You can configure your GPU instances with the specific models you need, and even choose the amount of storage, vCPUs, and network bandwidth to match your workload.
For example, if you’re developing a predictive model for global consumer preferences, Lambda Labs allows you to fine-tune your instance specifications to get the best possible performance.
Furthermore, Lambda Labs allows you to reserve clusters for long periods, which is great for users who need consistent access to GPU resources without worrying about fluctuating availability or pricing.
Google Colab:
Colab is much more streamlined in its approach, offering fewer customization options. The system is designed to get you up and running quickly, which is ideal for smaller projects.
While you can upgrade to more powerful GPUs in Colab’s Pro and Pro+ tiers, you won’t have the same level of control over your instance configurations. This can be limiting for users working on more advanced tasks, such as to train custom image models.
CUDO Compute delivers powerful and budget-friendly cloud GPU options specifically crafted to meet the demands of your AI and machine learning projects. Sign up now!
Specific Use Cases
Developing Custom Machine Learning Models
- Google Colab is useful for developing custom machine learning models, especially when leveraging Google’s integrated tools like the Natural Language API or Google Cloud’s Vision API.
- Lambda Labs, on the other hand, is better suited for more extensive and resource-demanding projects. Their on-demand H100 GPUs offer excellent performance for training transformer models or running industry-leading prediction accuracy tests.
Interactive and Real-Time AI Applications
- Google Colab offers good support for applications like interactive voice response systems or virtual agent services where the project demands occasional, high-performance compute resources but not continuous uptime.
- Lambda Labs, with their multi-node and multimodal capabilities, is a better fit for projects that require continuous, high-throughput, and dynamic and targeted recommendations systems. The high-speed networking and large GPU clusters make Lambda ideal for sophisticated AI use cases.
Lambda Labs vs Google Colab: Final Thoughts
Choosing between Google Colab and Lambda Labs ultimately depends on your specific needs:
Choose Google Colab if you’re looking for ease of use, no long-term commitment, and access to Google Cloud’s ecosystem for smaller AI projects, especially those involving data analysis, natural language understanding, or prototyping.
Choose Lambda Labs if you need dedicated, high-performance GPU clusters for training large models, want flexibility with pricing for on-demand or reserved usage, and need the most powerful GPUs available. Lambda is ideal for enterprises or developers working with a sophisticated collective intelligence network or a project that predicts global consumer preferences.
Additionally, when dealing with niche datasets, you may find the ability to identify domain specific entities to be critical in achieving the highest possible model performance. Consider which service aligns with your use cases in terms of flexibility, scalability, and support for specialized machine learning tasks.
For your AI and machine learning projects, look to CUDO Compute for strong and cost-effective cloud GPU solutions designed to fit your needs. Sign up now!