RunPod vs SageMaker: Which One Is Best for Seamless AI Model Deployment?
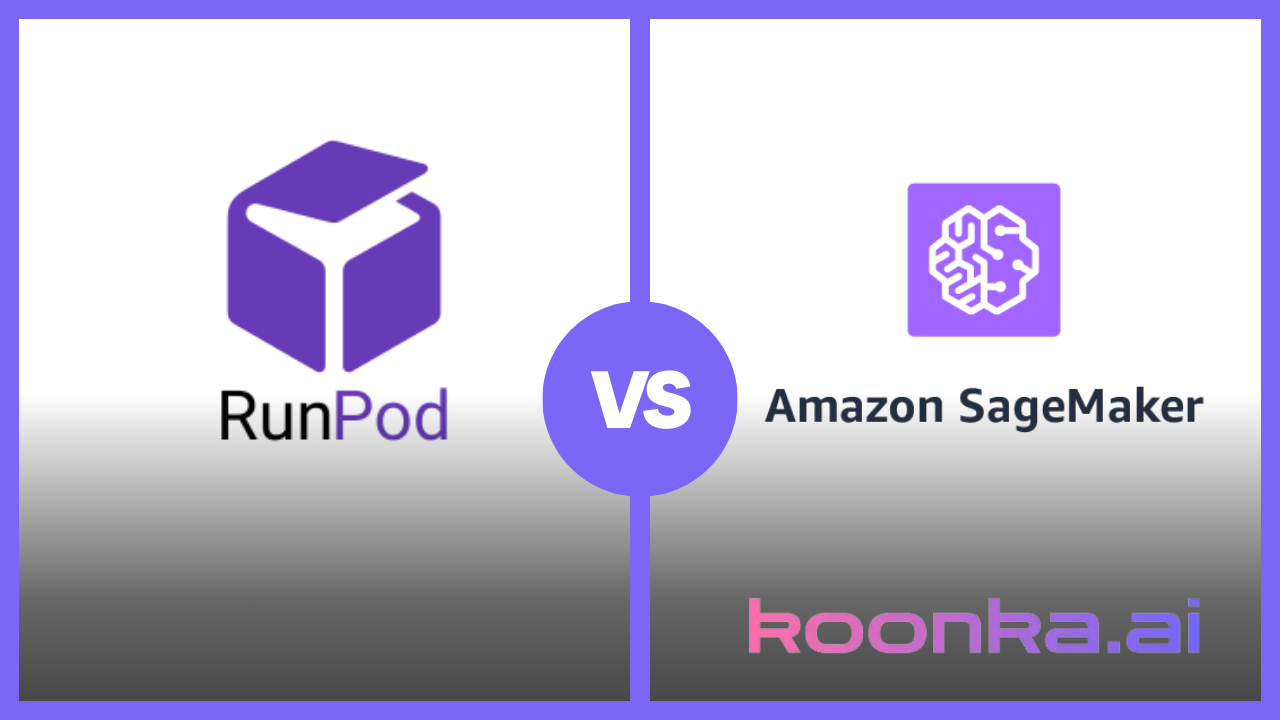
Trying to decide between RunPod and SageMaker for your AI workloads? We’ve got you covered. RunPod makes things super easy—spin up GPU pods in seconds, get instant hot reloads, and use pre-built templates to start running your models fast. It feels like working locally but with all the power of the cloud. On the flip side, SageMaker comes packed with built-in algorithms and serious integration with AWS, making it a go-to for large-scale machine-learning projects. Both platforms offer speed, scalability, and powerful GPUs. But which one’s the best fit for your needs? Let’s break down the RunPod vs SageMaker comparison so that you can make the right choice!
Table of Contents
RunPod vs SageMaker: The Main Difference
RunPod offers a more flexible and cost-effective solution with support for both NVIDIA and AMD GPUs, while SageMaker is deeply integrated into the AWS ecosystem, providing enterprise-level scalability, advanced machine learning features, and seamless cloud-native workflows tailored for large-scale AI deployments.
Affiliate Disclosure
We prioritize transparency with our readers. If you purchase through our affiliate links, we may earn a commission at no additional cost to you. These commissions enable us to provide independent, high-quality content to our readers. We only recommend products and services that we personally use or have thoroughly researched and believe will add value to our audience.
GPU Compute Comparison
Let’s break down what both providers offer in terms of GPU instances, architecture, pricing, and features, so you can make an informed decision.
Searching for budget-friendly, high-performance GPUs? CUDO Compute offers on-demand rentals for leading NVIDIA and AMD graphics cards. Sign up now!
To learn more about CUDO Compute, kindly watch the following video:
GPU Instances: A Variety to Choose From
Both RunPod and SageMaker offer a range of powerful GPUs that cater to AI model training, deep learning tasks, and machine learning workflows. However, the variety and flexibility they offer differ significantly.
RunPod GPU Instances:
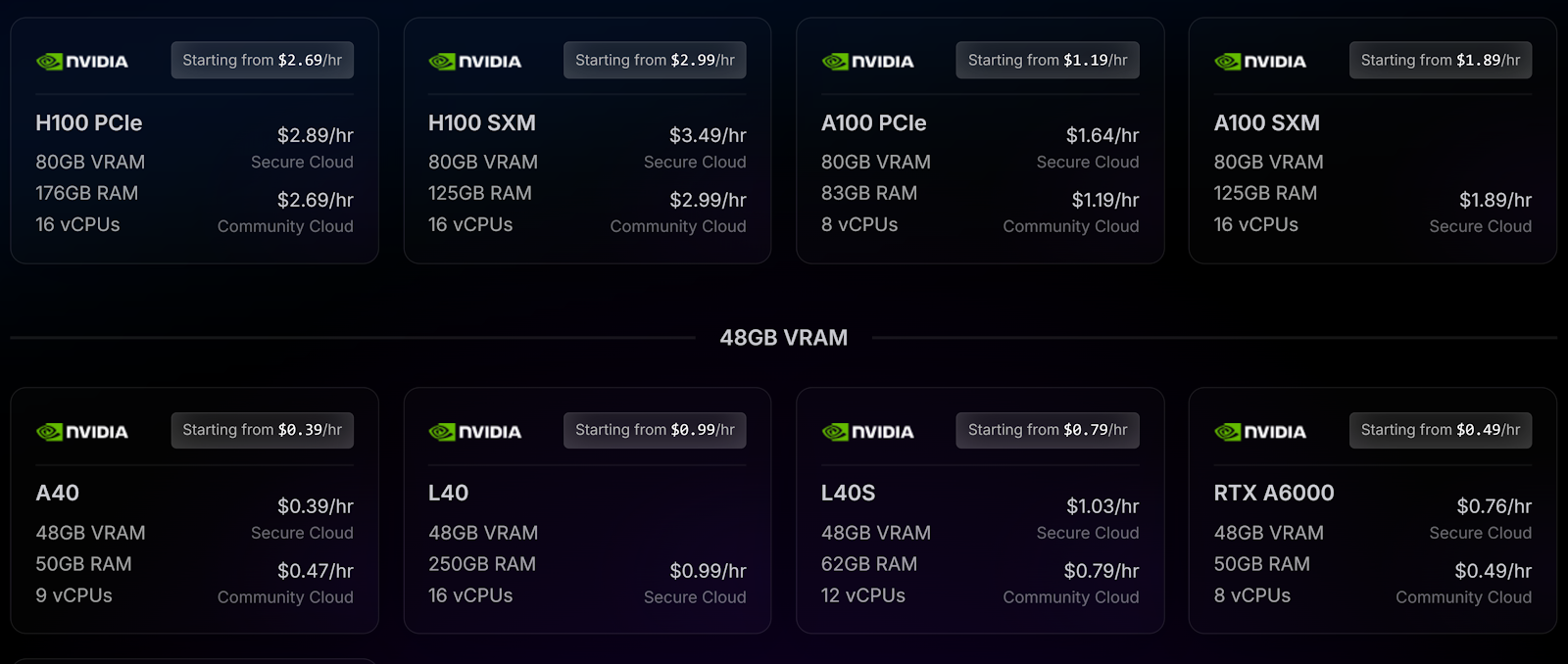
NVIDIA A40:
The NVIDIA A40 is designed for smaller-scale machine learning tasks, AI inference, and rendering workloads. With 48GB of VRAM and 50GB of RAM, it’s ideal for tasks that don’t require massive computing power but still need efficient performance.
Its cost-effective pricing makes it a great choice for startups and individuals working on smaller projects, like fine-tuning existing models or running inference on neural networks.
Additionally, its lower power consumption compared to other high-end GPUs makes it an appealing option for sustained workloads without breaking the bank.
NVIDIA H100 PCIe:
The NVIDIA H100 PCIe is a top-tier GPU for handling the most demanding AI tasks, such as training complex machine learning models, deep learning tasks, and large-scale neural networks.
With 80GB of VRAM and 176GB of RAM, it offers exceptional performance and memory bandwidth, allowing data scientists to work on large datasets and advanced AI models without bottlenecks.
Priced at $2.69/hr, it’s an excellent choice for enterprises or researchers looking for scalable infrastructure to train AI models, run simulations, or even deploy GPU instances for high-end computing power.
NVIDIA A100 SXM:
The NVIDIA A100 SXM is designed for machine learning, AI model training, and inference at a larger scale. With 80GB of VRAM and 125GB of RAM, it provides enough memory and bandwidth for handling more intensive computing tasks like training large neural networks and deep learning applications.
At $1.94/hr, it’s a cost-effective solution for users requiring high computational power without the price tag of higher-end GPUs like the H100.
Its versatility and performance make it ideal for both research and production environments where running multiple models simultaneously is crucial.
AMD MI300X:
The AMD MI300X, available for $3.99/hr, is RunPod’s premium offering for those preferring AMD hardware.
With an impressive 192GB of VRAM and 283GB of RAM, it is specifically built for data-intensive workloads, including AI training, machine learning, and complex simulations.
Its high memory capacity allows it to handle the heaviest AI models and large datasets with ease, making it perfect for professionals working in industries like research, data science, and emerging technologies.
It’s also a great choice for those building custom models that require a balance of both computing power and memory.
Boost your AI and machine learning projects with CUDO Compute’s robust cloud GPUs. Begin scaling your initiatives today— Sign up now!
SageMaker GPU Instances:
NVIDIA H100 (P5):
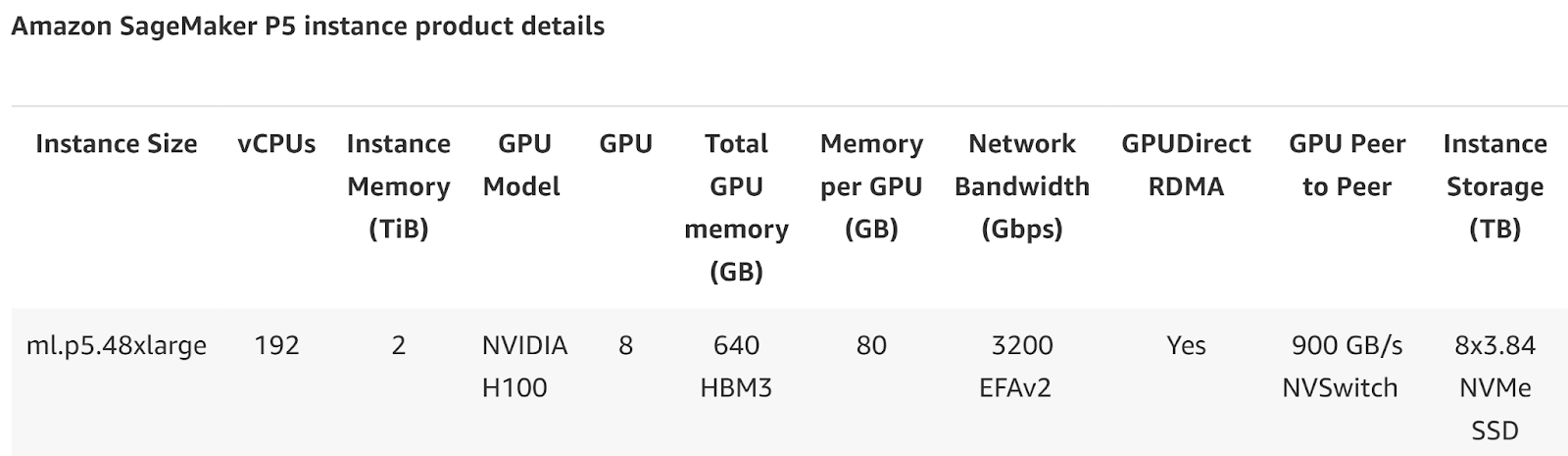
The NVIDIA H100 GPUs available on SageMaker’s ml.p5.48xlarge instances are designed for cutting-edge AI workloads. With 8 H100 GPUs and a combined 640GB of HBM3 memory, this instance is ideal for large-scale deep learning and generative AI models.
It offers immense computational power, making it perfect for tasks like training large language models, image generation, or running simulations that require high memory bandwidth and GPU resources.
At $3.99/hr, this instance is suited for enterprises and researchers who need to process large datasets quickly and efficiently, without compromising on performance.
NVIDIA A100 (P4d):
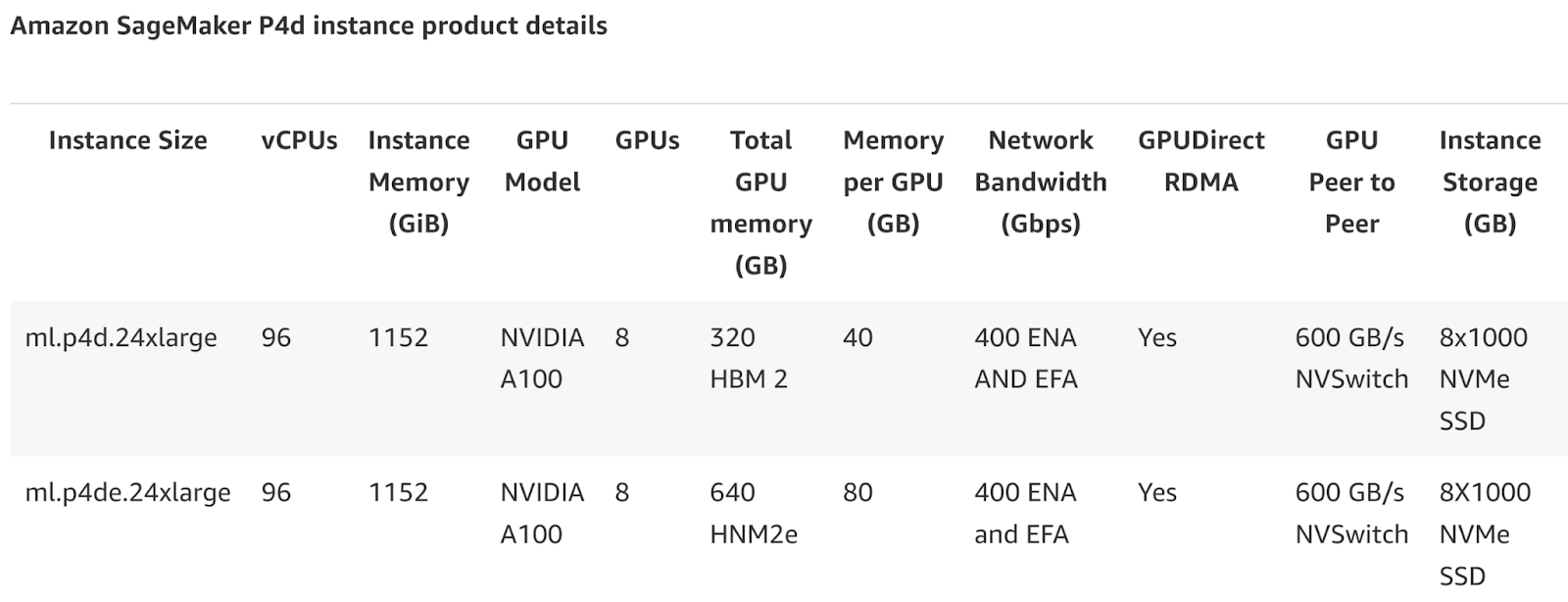
The NVIDIA A100 on SageMaker’s ml.p4d.24xlarge instance is built for deep learning tasks at scale. With 8 A100 GPUs and 320GB of VRAM, it is optimized for handling high-performance computing, AI training, and massive datasets.
Each GPU offers excellent memory bandwidth and performance, making it a top choice for users needing to train neural networks, perform large-scale data processing, or deploy AI models.
At $2.99/hr, it’s a more affordable option for users requiring significant GPU power, but without the same memory capabilities as the H100 instances.
NVIDIA V100 (P3):
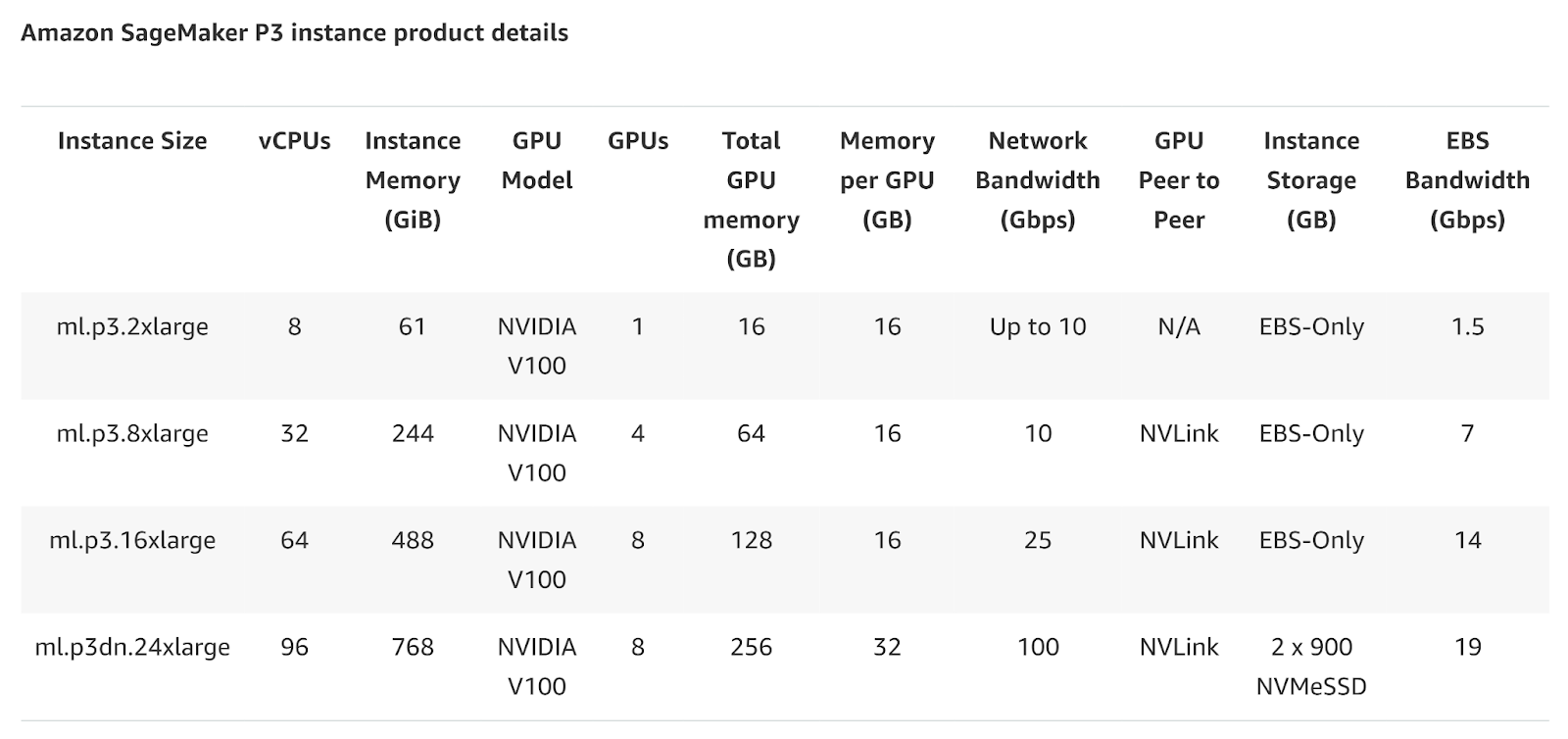
SageMaker’s NVIDIA V100 GPUs, available in the ml.p3 instance family, are tailored for mid-tier machine learning and AI workloads.
With 16GB of VRAM per GPU, it strikes a balance between cost and performance, making it suitable for users working on AI model development, data processing, or medium-scale neural network training.
It’s ideal for users who don’t need the heavy-duty performance of the A100 or H100 but still require solid computing power for training models, performing inference, or running simulations.
This instance is perfect for startups or small teams focused on AI research at a lower cost.
Need flexible and scalable GPU cloud services for AI or rendering? CUDO Compute offers dependable solutions at competitive prices. Sign up now!
GPU Architecture: What’s Under the Hood
The architecture of the GPUs on both platforms is critical when deciding based on performance needs. NVIDIA’s Ampere and Hopper architectures dominate the landscape for high-end computing, while AMD’s MI series GPUs offer a competitive alternative.
RunPod GPU Architecture:
- NVIDIA Ampere: The A100 and A40 models run on NVIDIA’s Ampere architecture, which excels in both training and inference tasks for machine learning models.
- NVIDIA Hopper: The H100, which is based on NVIDIA’s Hopper architecture, is designed specifically for the next generation of neural networks and artificial intelligence models.
- AMD MI300X: RunPod offers AMD’s MI300X, built on the advanced CDNA architecture. It provides impressive memory bandwidth, making it perfect for handling large AI and machine learning workloads.
SageMaker GPU Architecture:
- NVIDIA Hopper: SageMaker’s H100 instances, part of the P5 series, leverage the Hopper architecture for demanding AI workloads, offering faster computation for deep learning tasks.
- NVIDIA Ampere: SageMaker’s A100 instances also operate on the Ampere architecture, which is excellent for general-purpose machine learning model training and inference.
Pricing Structure: Cost-Effective Solutions for Every Budget
One of the major deciding factors between these platforms is the pricing structure. Let’s take a closer look at the pricing of GPU instances on both services.
RunPod Pricing:
- NVIDIA A40: $0.39/hr – This is RunPod’s most affordable GPU, making it a great option for small AI inference tasks and low-intensity GPU usage.
- NVIDIA H100: $2.69/hr for the PCIe version, making it a bit cheaper than the same offering on SageMaker.
- AMD MI300X: At $3.99/hr, this is RunPod’s most expensive option, ideal for users who need massive computing power for long-running deep learning tasks.
SageMaker Pricing:
- NVIDIA H100 (P5): Starting at approximately $3.99/hr, SageMaker’s H100 instance is similar in price to RunPod but offers more GPU memory (640GB HBM3).
- NVIDIA A100 (P4d): Priced at $2.99/hr, SageMaker’s A100 instances are ideal for large-scale machine learning model training and deep learning inference tasks.
SageMaker has a slightly higher price range for similar GPUs, but it provides more advanced infrastructure, such as 640GB of memory for its H100 GPUs.
Gain immediate access to NVIDIA and AMD GPUs on-demand with CUDO Compute—ideal for AI, machine learning, and high-performance computing projects. Sign up now!
Use Cases for Each Platform
Different GPUs are designed to tackle different kinds of workloads. Understanding where each GPU shines is essential.
RunPod Use Cases:
- A40 & A100 GPUs: Excellent for medium-sized AI model training and stable diffusion tasks.
- H100: The ideal choice for training large language models (LLMs) and neural networks.
- MI300X: Best suited for cutting-edge AI research and enterprise-level deep learning tasks.
SageMaker Use Cases:
- P3 Instances (V100 GPUs): These are great for small to medium machine learning models.
- P5 Instances (H100 GPUs): These are the top choice for massive machine learning workloads, such as those required by enterprises dealing with massive datasets.
Scalability and Infrastructure
Scalable infrastructure is key for growing AI workloads, especially as model complexity increases.
RunPod Scalability:
- Autoscaling in Seconds: RunPod offers fast autoscaling, making it easy to go from 0 to 100 GPUs in seconds. This is a huge plus if your workload fluctuates.
- Serverless Options: RunPod’s serverless GPU workers offer a flexible way to handle unpredictable AI inference workloads. You only pay when your endpoint receives a request, and you can auto-scale GPU instances based on demand.
SageMaker Scalability:
- Deep Integration with AWS: SageMaker is integrated with the broader AWS ecosystem, enabling users to leverage existing Kubernetes clusters and other AWS services. This is ideal for businesses already invested in AWS infrastructure.
- Elastic Inference: SageMaker offers elastic inference, which automatically scales GPU usage based on the needs of your machine learning models. This allows for more cost-effective use of GPU resources.
Storage and Networking
Both platforms provide storage solutions and high-speed networking, but SageMaker’s integration with AWS gives it an edge in network performance.
RunPod Storage and Networking:
- NVMe SSD Storage: RunPod offers NVMe-backed storage with up to 100Gbps throughput, making it ideal for AI models requiring fast data access.
- Persistent Network Volumes: RunPod supports network storage volumes of up to 100TB+, perfect for long-term storage needs.
SageMaker Storage and Networking:
- EBS and NVMe Storage: SageMaker offers 8×3.84TB NVMe SSD storage in its P5 instances, ideal for large-scale machine learning tasks requiring fast read/write speeds.
- High Bandwidth: SageMaker’s H100 instances offer 900GB/s NVSwitch, providing ultra-fast interconnects between GPUs, which is crucial for large-scale neural network training.
Model Training: Speed and Efficiency
Both platforms are designed to handle heavy AI model training workloads, but they offer different tools to make model training as efficient as possible.
RunPod Model Training:
- Serverless AI Training: RunPod’s serverless AI training allows for efficient autoscaling, reducing costs for long-running training tasks.
- Zero Overhead Deployment: With RunPod, you can deploy your models and scale them without worrying about operational overhead. This lets you focus on model training while RunPod manages the infrastructure.
SageMaker Model Training:
- Built-In Algorithms: SageMaker comes with built-in algorithms for common machine learning tasks, reducing the need for custom models from scratch.
- Real-Time Debugging: SageMaker provides real-time debugging during model training, making it easier to troubleshoot issues and optimize GPU usage.
Cost-Effective Solutions for AI Inference
When it comes to AI inference, both RunPod and SageMaker offer cost-effective solutions tailored for data scientists and AI developers, although their pricing structures and feature sets differ significantly. These platforms are designed to maximize efficiency while minimizing costs, making them attractive options for various AI projects.
RunPod Inference Solutions:
RunPod leverages innovative technology to enhance its inference offerings. One standout feature is Flashboot Technology, which significantly reduces cold-start times to less than 250ms. This rapid response capability is essential for real-time applications, such as chatbots or recommendation engines, where delays can adversely affect user experience.
Additionally, RunPod adopts a Pay-Per-Request pricing model for its serverless endpoints. This structure charges users based on the number of requests processed, allowing for greater cost flexibility.
For developers who may have variable traffic patterns or seasonal spikes in usage, this model ensures that they only pay for the actual resources consumed, avoiding the fixed costs associated with traditional server models.
SageMaker Inference Solutions:
On the other hand, SageMaker offers its own innovative approach to cost-effective AI inference through Elastic Inference. This feature allows users to adjust GPU power dynamically based on workload demands.
By scaling GPU resources up or down according to the needs of specific inference tasks, SageMaker helps optimize overall costs.
This adaptability is particularly useful for projects that experience fluctuating demands, as it prevents over-provisioning of resources while ensuring that performance remains high when needed.
Support for Emerging Technologies
Both platforms are positioned to support emerging technologies, especially in the realm of AI and deep learning.
RunPod:
RunPod’s flexibility in supporting both NVIDIA and AMD GPUs makes it ideal for developers working on cutting-edge technologies, from stable diffusion to LLMs like GPT and Llama. Its cost-effective pricing is attractive to startups and research teams pushing the boundaries of AI.
SageMaker:
With deeper AWS integration and built-in support for Kubernetes, SageMaker like IBM Cloud and other big tech companies is better suited for enterprises leveraging cloud-native solutions. Its infrastructure is designed to handle the demands of large-scale AI workloads with the necessary speed, reliability, and scalability.
RunPod vs SageMaker: The Bottom Line
Both RunPod and SageMaker offer competitive GPU compute solutions. The right choice depends on your specific needs. RunPod provides a more flexible, cost-effective option for developers and researchers working with both NVIDIA and AMD hardware. On the other hand, SageMaker’s integration with the AWS ecosystem and advanced features like elastic inference and built-in algorithms make it a better choice for enterprises already invested in the AWS cloud.
CUDO Compute provides robust and affordable cloud GPU solutions tailored for your AI and machine learning needs. Sign up now!